High-dimensional complex system uncertainty analysis method based on statistical machine learning
A statistical machine learning and uncertainty technology, applied in the field of uncertainty analysis of high-dimensional complex systems based on statistical machine learning, can solve the problems of insignificant training effect, increased model burden, model overfitting, etc. The effect of reducing the amount of calculation, reducing the number of dimensions
- Summary
- Abstract
- Description
- Claims
- Application Information
AI Technical Summary
Problems solved by technology
Method used
Image
Examples
Embodiment Construction
[0020] Embodiments of the present invention are described in detail below, examples of which are shown in the drawings, wherein the same or similar reference numerals designate the same or similar elements or elements having the same or similar functions throughout. The embodiments described below by referring to the figures are exemplary and are intended to explain the present invention and should not be construed as limiting the present invention.
[0021] The method for analyzing uncertainty of a high-dimensional complex system based on statistical machine learning according to an embodiment of the present invention will be described below with reference to the accompanying drawings.
[0022] figure 1 It is a flowchart of an uncertainty analysis method for high-dimensional complex systems based on statistical machine learning according to an embodiment of the present invention.
[0023] Such as figure 1 As shown, the high-dimensional complex system uncertainty analysis me...
PUM
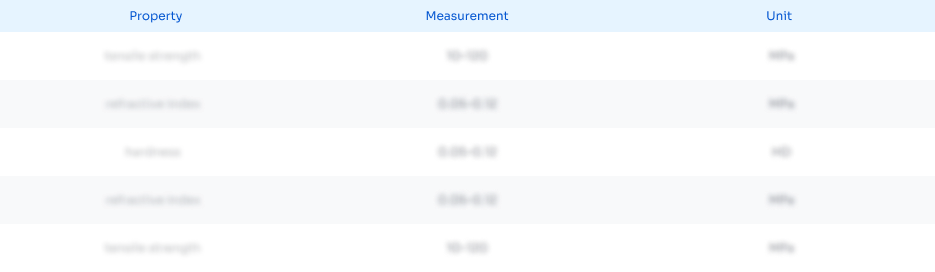
Abstract
Description
Claims
Application Information
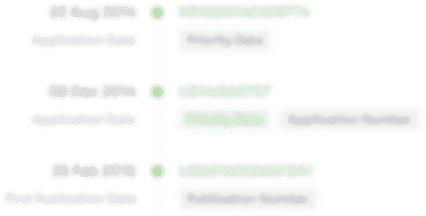
- R&D
- Intellectual Property
- Life Sciences
- Materials
- Tech Scout
- Unparalleled Data Quality
- Higher Quality Content
- 60% Fewer Hallucinations
Browse by: Latest US Patents, China's latest patents, Technical Efficacy Thesaurus, Application Domain, Technology Topic, Popular Technical Reports.
© 2025 PatSnap. All rights reserved.Legal|Privacy policy|Modern Slavery Act Transparency Statement|Sitemap|About US| Contact US: help@patsnap.com