Disease factor extraction method based on improved PSO-BP neural network and Bayesian method
A PSO-BP, BP neural network technology, applied in the design of big data technology and medical fields, can solve the problems of network non-convergence, heavy learning burden, and low training efficiency, so as to improve the convergence accuracy and generalization ability, and solve the impact size Effects of inaccuracy, efficiency, and high data utilization
- Summary
- Abstract
- Description
- Claims
- Application Information
AI Technical Summary
Problems solved by technology
Method used
Image
Examples
Embodiment
[0090] The present invention is based on the disease factor extraction method of improved PSO-BP neural network and Bayesian method, comprises the following contents:
[0091] 1. By introducing an adaptive weight strategy, optimize the particle swarm optimization algorithm (PSO), specifically:
[0092] The position formula of particle swarm algorithm:
[0093] x id (t+1)=X id (t)+V id (t+1)
[0094] Introduce the adaptive inertia weight w(t) in the speed formula:
[0095] V id (t+1)=w(t)V id (t)+c 1 r 1 ·(P best -X id (t))+c 2 r 2 ·(G best -X id (t))
[0096] Among them, the adaptive inertia weight w(t) is:
[0097]
[0098] In the formula, V id and x id are the velocity and position of the i-th particle, respectively; P best is the best position experienced by the i-th particle in the iteration, i.e. the optimal solution, G best is the optimal position in the particle swarm; c 1 、c 2Both are acceleration factors, usually c 1 = c 2 = 1.5; r 1 、r 2 ...
PUM
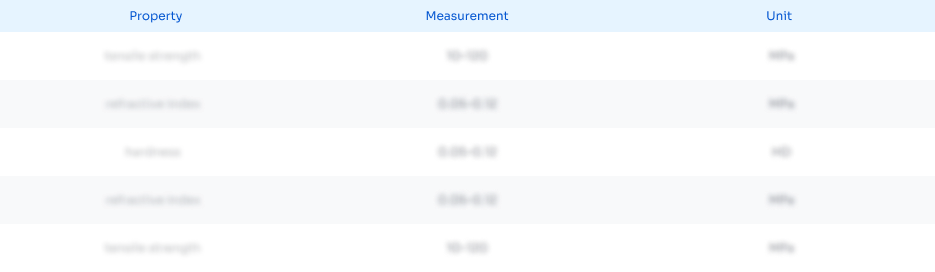
Abstract
Description
Claims
Application Information
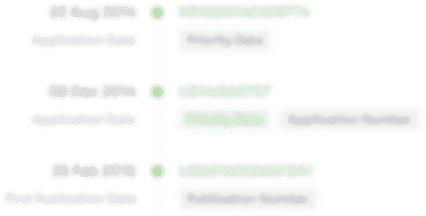
- R&D Engineer
- R&D Manager
- IP Professional
- Industry Leading Data Capabilities
- Powerful AI technology
- Patent DNA Extraction
Browse by: Latest US Patents, China's latest patents, Technical Efficacy Thesaurus, Application Domain, Technology Topic, Popular Technical Reports.
© 2024 PatSnap. All rights reserved.Legal|Privacy policy|Modern Slavery Act Transparency Statement|Sitemap|About US| Contact US: help@patsnap.com