Binary neural network acceleration method and system based on FPGA
A binary neural network and acceleration system technology, which is applied in FPGA-based binary neural network acceleration methods and systems, can solve the problems of convolutional neural network deployment difficulties, difficulty in achieving real-time effects, and consumption of computing resources. The effect of reducing communication costs, increasing convolution calculation speed, and improving detection speed
- Summary
- Abstract
- Description
- Claims
- Application Information
AI Technical Summary
Problems solved by technology
Method used
Image
Examples
Embodiment Construction
[0039] In order to make the object, technical solution and advantages of the present invention clearer, the present invention will be further described in detail below in conjunction with the accompanying drawings and embodiments. It should be understood that the specific embodiments described here are only used to explain the present invention, not to limit the present invention.
[0040] In addition, the technical features involved in the various embodiments of the present invention described below can be combined with each other as long as they do not constitute a conflict with each other. The present invention will be further described in detail below in combination with specific embodiments.
[0041] figure 1 It is a structural schematic diagram of an FPGA-based binary neural network acceleration system according to an embodiment of the present invention. Such as figure 1 As shown, a binary neural network acceleration system based on FPGA, the system includes a convolu...
PUM
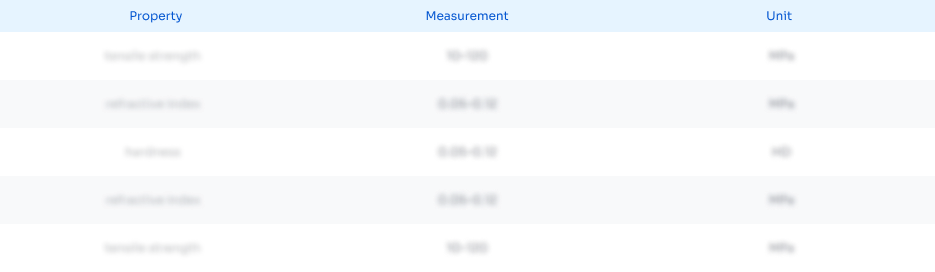
Abstract
Description
Claims
Application Information
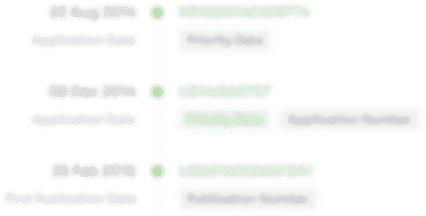
- R&D Engineer
- R&D Manager
- IP Professional
- Industry Leading Data Capabilities
- Powerful AI technology
- Patent DNA Extraction
Browse by: Latest US Patents, China's latest patents, Technical Efficacy Thesaurus, Application Domain, Technology Topic, Popular Technical Reports.
© 2024 PatSnap. All rights reserved.Legal|Privacy policy|Modern Slavery Act Transparency Statement|Sitemap|About US| Contact US: help@patsnap.com