Face recognition method based on semi-supervised training
A face recognition, semi-supervised technology, applied in the field of computer vision, can solve the problems of inconsistent numbers, low accuracy of clustering algorithms, and unbalanced categories, and achieve good performance improvement, good universality, and improved performance.
- Summary
- Abstract
- Description
- Claims
- Application Information
AI Technical Summary
Problems solved by technology
Method used
Image
Examples
Embodiment
[0044] This embodiment is the overall process and network structure of the face recognition model using semi-supervised training.
[0045] A face recognition method based on semi-supervised training, such as figure 1 shown, including the following steps:
[0046] Step 1: Obtain and preprocess training data;
[0047] Such as figure 2 As shown, for the unlabeled data, crawl the face picture from the Internet, use the MTCNN face detector to detect the face, and get the face rectangle frame and five key points; according to the face rectangle frame and five key points, Use OpenCV's warpAffine function for face alignment, and the size of the aligned image is 112×112; for labeled data, use an existing face recognition dataset, such as MS1M released by Microsoft, to perform face detection like unlabeled data Align with and the size of the aligned image is 112×112.
[0048] Step 2: Design the network structure model;
[0049] Such as image 3 As shown, the network structure mod...
PUM
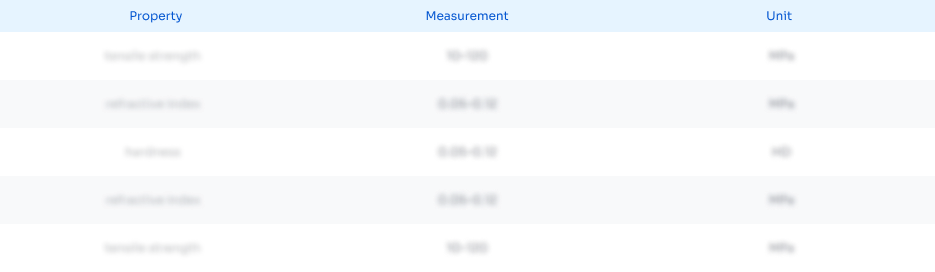
Abstract
Description
Claims
Application Information
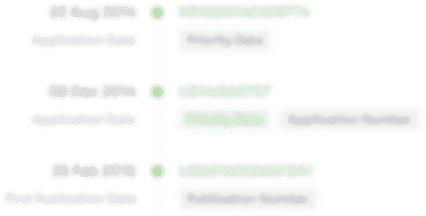
- R&D
- Intellectual Property
- Life Sciences
- Materials
- Tech Scout
- Unparalleled Data Quality
- Higher Quality Content
- 60% Fewer Hallucinations
Browse by: Latest US Patents, China's latest patents, Technical Efficacy Thesaurus, Application Domain, Technology Topic, Popular Technical Reports.
© 2025 PatSnap. All rights reserved.Legal|Privacy policy|Modern Slavery Act Transparency Statement|Sitemap|About US| Contact US: help@patsnap.com