Power system short-term load prediction method based on time convolution network
A short-term load forecasting, convolutional network technology, applied in the field of power system and automation, can solve the problems of long time, large memory, occupation, etc., to simplify the learning objectives and difficulty, reduce time and hardware requirements.
- Summary
- Abstract
- Description
- Claims
- Application Information
AI Technical Summary
Problems solved by technology
Method used
Image
Examples
Embodiment 1
[0035] Such as figure 1 , figure 2 As shown, a short-term load forecasting method for power systems based on temporal convolutional networks includes the following steps:
[0036] S1: Collect historical load data and preprocess the data;
[0037] S2: Construct a causal dilated convolution model, and input the preprocessed data into two different causal dilated convolution models for convolution processing;
[0038] S3: Connect the two results processed by the causal dilated convolution model to form a residual block;
[0039] S4: Stack the residual blocks to obtain a temporal convolutional network;
[0040] S5: Use the temporal convolutional network to perform full convolutional layer calculations to predict future power load demand.
[0041] More specifically, in step S1, the data preprocessing includes missing data completion processing and normalization processing; wherein:
[0042] The missing data completion process is to use the average adjacent load to replace out...
Embodiment 2
[0055] More specifically, on the basis of Example 1, in order to verify the scientificity and reliability of the method proposed in this paper, the experiment in this paper uses the electric load data of Toronto in 2016 as a data set, and finally compares the experimental results with the classic SVRM model and The comparative analysis of the prediction results of the LSTM model shows that the model proposed in this paper has achieved better prediction accuracy.
[0056]In the specific implementation process, 90% of the data set is divided into training sets, and 10% of the data set is divided into test sets; the workstation is selected as the hardware platform: including Intel Core i7-8700k processor, 32GB memory, 256GB solid state drive, GTX1080TI 11G graphics card. The software framework structure is the TensorFlow framework based on Keras deep learning. Keras provides a concise and consistent programming interface, which can help users quickly understand the neural network...
PUM
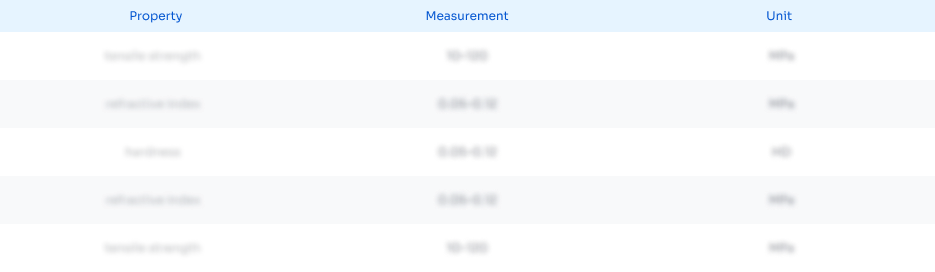
Abstract
Description
Claims
Application Information
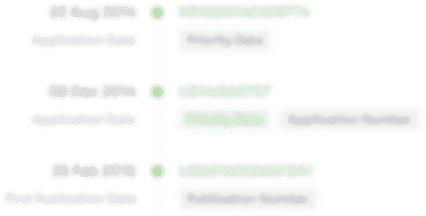
- R&D Engineer
- R&D Manager
- IP Professional
- Industry Leading Data Capabilities
- Powerful AI technology
- Patent DNA Extraction
Browse by: Latest US Patents, China's latest patents, Technical Efficacy Thesaurus, Application Domain, Technology Topic, Popular Technical Reports.
© 2024 PatSnap. All rights reserved.Legal|Privacy policy|Modern Slavery Act Transparency Statement|Sitemap|About US| Contact US: help@patsnap.com