Unmanned aerial vehicle trajectory optimization method and device based on deep reinforcement learning and unmanned aerial vehicle
A technology of reinforcement learning and trajectory optimization, applied in the field of drones, can solve problems such as dimension explosion, information loss, and limited flight action plans, and achieve the effect of avoiding losses and improving energy efficiency
- Summary
- Abstract
- Description
- Claims
- Application Information
AI Technical Summary
Problems solved by technology
Method used
Image
Examples
Embodiment 1
[0053] Deep reinforcement learning technology is a machine learning technique that combines reinforcement learning and deep neural networks. Specifically, reinforcement learning individuals collect reward information for taking different actions in different environmental states by interacting with the environment, and inductively learn the optimal behavior strategy based on the collected data, so as to obtain the ability to adapt to the unknown dynamic environment . The deep neural network can significantly improve the generalization ability of the algorithm in high-dimensional state space and high-dimensional action space, so as to obtain the ability to adapt to more complex environments.
[0054] Embodiment 1 of the present invention provides a UAV trajectory optimization method based on deep reinforcement learning, such as figure 1 As shown, the method includes the following steps:
[0055] S101, pre-constructing a deep reinforcement learning network based on a PPO algor...
Embodiment 2
[0065] Embodiment 2 of the present invention provides another embodiment of a UAV trajectory optimization method based on deep reinforcement learning.
[0066] In Embodiment 2 of the present invention, the PPO algorithm adopts the deep reinforcement learning structure of the actor-critic (Actor-Critic) framework, and is composed of two networks: the action network and the evaluation network: the action network uses the PPO algorithm and the deep neural network to fit the strategy function, decision-making action; the evaluation network uses the deep neural network to fit the state-value function and optimize the policy parameters. The overall structure and related data interaction of the optimization method provided by Embodiment 2 of the present invention are as follows: figure 2 shown.
[0067] In this embodiment, the UAV communication scenario used is that a single UAV base station provides services for multiple fixed IoT devices, and the IoT devices are randomly activate...
Embodiment 3
[0075] Embodiment 3 of the present invention provides a preferred embodiment of the UAV trajectory optimization method based on deep reinforcement learning. Through this embodiment, the UAV communication modeling method used in the present invention and the UAV energy-efficient trajectory based on deep reinforcement learning The optimization method is described in further detail.
[0076] The UAV communication model established in this embodiment considers a scenario where a UAV provides delay-tolerant services for N terrestrial Internet of Things devices. The Internet of Things devices are randomly distributed and their positions are fixed, and data is collected and transmitted periodically or randomly. to drones. The goal is to optimize the flight trajectory of the UAV to maximize the cumulative energy efficiency under energy-limited conditions. In order to achieve this goal, the UAV should be able to detect its own remaining energy and determine the optimal return charging...
PUM
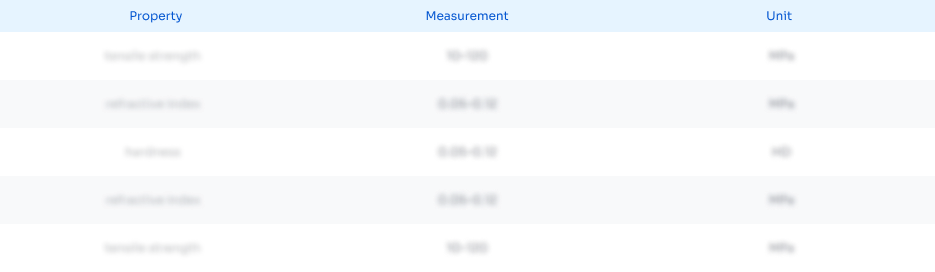
Abstract
Description
Claims
Application Information
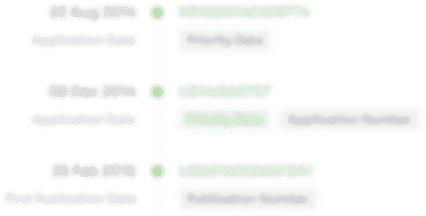
- R&D Engineer
- R&D Manager
- IP Professional
- Industry Leading Data Capabilities
- Powerful AI technology
- Patent DNA Extraction
Browse by: Latest US Patents, China's latest patents, Technical Efficacy Thesaurus, Application Domain, Technology Topic, Popular Technical Reports.
© 2024 PatSnap. All rights reserved.Legal|Privacy policy|Modern Slavery Act Transparency Statement|Sitemap|About US| Contact US: help@patsnap.com