Feature-level fusion method for multi-modal emotion detection
A feature-level fusion and multi-modal technology, applied in character and pattern recognition, special data processing applications, biological neural network models, etc., to achieve the effect of simple operation, stable effect and fast operation speed
- Summary
- Abstract
- Description
- Claims
- Application Information
AI Technical Summary
Problems solved by technology
Method used
Image
Examples
Embodiment Construction
[0039] The present invention will be described in detail below in conjunction with the accompanying drawings, so that the advantages and features of the present invention can be more easily understood by those skilled in the art, so as to define the protection scope of the present invention more clearly.
[0040] refer to figure 1 and figure 2 , a feature-level fusion method for multimodal emotion detection, comprising the following steps:
[0041] Step 1: Obtain the transcript of its text form from the public IEMOCAP multimodal dataset. The transcript S is a sentence composed of n words, that is, S=[w 1 ,w 2 ,...,w n ];
[0042] Step 2: According to the existing fast text embedding dictionary, the initial one-hot vector word W with dimension V i Embed into a low-dimensional real-valued vector to obtain a vector sequence X;
[0043] By formula: Embedding the word, transforming the sentence S into a vector sequence X=[x 1 ,x 2 ,...x n ];
[0044] Step 3: Apply a si...
PUM
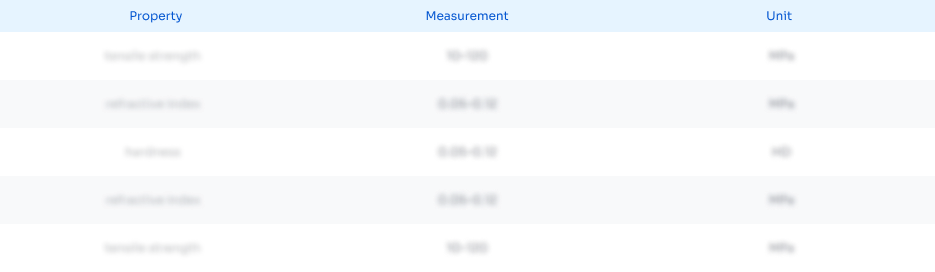
Abstract
Description
Claims
Application Information
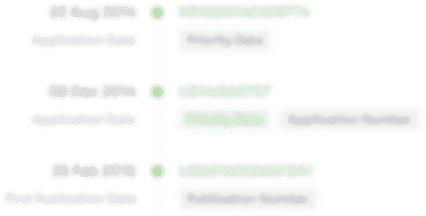
- R&D Engineer
- R&D Manager
- IP Professional
- Industry Leading Data Capabilities
- Powerful AI technology
- Patent DNA Extraction
Browse by: Latest US Patents, China's latest patents, Technical Efficacy Thesaurus, Application Domain, Technology Topic, Popular Technical Reports.
© 2024 PatSnap. All rights reserved.Legal|Privacy policy|Modern Slavery Act Transparency Statement|Sitemap|About US| Contact US: help@patsnap.com