Solar cell panel defect detection method based on multi-scale joint convolutional neural network
A solar panel and convolutional neural network technology, applied in neural learning methods, biological neural network models, neural architectures, etc., can solve the problems of a set of parameters that are difficult to use, single function, and few detectable categories, etc., to achieve strong generality The effect of improving the stability and anti-interference ability, improving network efficiency, improving scale invariance and classification accuracy
- Summary
- Abstract
- Description
- Claims
- Application Information
AI Technical Summary
Problems solved by technology
Method used
Image
Examples
Embodiment 1
[0045] The first step is to collect solar panel image samples and data processing:
[0046] Collect image samples of solar panels and divide them into qualified samples and defect samples. Defect samples include defect samples with solid black, hidden cracks, broken grids, shadows and open welding defects. When the qualified sample data is more than the defective sample data , it is necessary to resample the solar panel image sample data set, that is, to perform data enhancement on less defective sample data by zooming, shifting, flipping, changing the brightness of the picture, and adding Gaussian noise after mean filtering, and for a large number of qualified samples The data randomly deletes a part of the image sample data to balance the collected solar panel image sample data, thereby completing the collection of solar panel image samples and data processing;
[0047] figure 1 It shows that the types of solar panel image samples collected in this embodiment include quali...
Embodiment 2
[0072] Except that in the second step of solar panel image preprocessing, the finally obtained training sample set has 2800 samples, and the test sample set has 1000 samples, other steps are the same as in embodiment 1.
Embodiment 3
[0074] Except that in the second step of solar panel image preprocessing, the finally obtained training sample set has 3200 samples, and the test sample set has 1400 samples, other steps are the same as in embodiment 1.
[0075] In the above-mentioned embodiment, described NVIDIA GTX965M graphics card, Intel's 6th generation i7 processor and ReLU activation function are well known in the art, and the various acquisitions mentioned, data processing, training, fusion, classification and testing operations are all It is within the grasp of those skilled in the art.
PUM
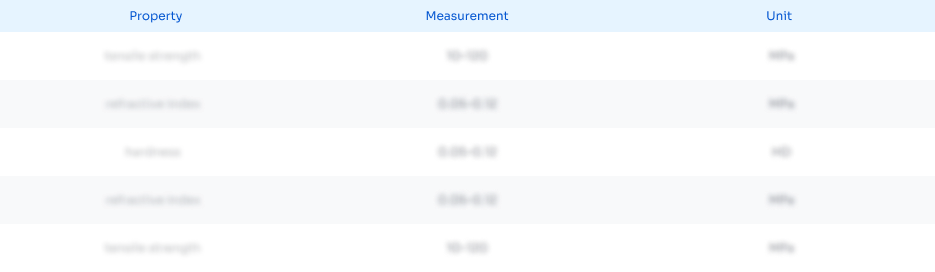
Abstract
Description
Claims
Application Information
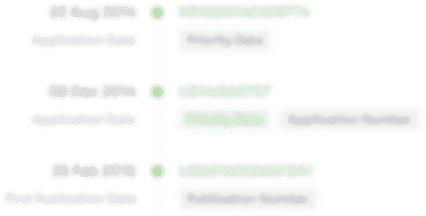
- R&D
- Intellectual Property
- Life Sciences
- Materials
- Tech Scout
- Unparalleled Data Quality
- Higher Quality Content
- 60% Fewer Hallucinations
Browse by: Latest US Patents, China's latest patents, Technical Efficacy Thesaurus, Application Domain, Technology Topic, Popular Technical Reports.
© 2025 PatSnap. All rights reserved.Legal|Privacy policy|Modern Slavery Act Transparency Statement|Sitemap|About US| Contact US: help@patsnap.com