Noise-like data low-frequency oscillation identification method based on double-covariance random subspace
A random subspace, low-frequency oscillation technology, applied to computer components, character and pattern recognition, instruments, etc.
- Summary
- Abstract
- Description
- Claims
- Application Information
AI Technical Summary
Problems solved by technology
Method used
Image
Examples
Embodiment Construction
[0067] The present invention will be further described below in conjunction with specific examples.
[0068] Such as figure 1 As shown, the method for identifying low-frequency oscillations of noise-like data based on double-covariance random subspaces provided in this embodiment includes the following steps:
[0069] 1) The random subspace of double covariance is used to process the power system noise-like signal, and the poles of two groups of characteristics are obtained, which is defined as the verification group H 1 and reference group H 2 , the specific steps are as follows:
[0070] 1.1) The random subspace of single covariance is used to process noise-like data, and the process is as follows:
[0071] 1.1.1) Construct two Hankel matrices with different numbers of rows and columns from the output of the system, where the Hankel matrix is defined as follows:
[0072]
[0073]
[0074]
[0075] Among them, a and b are the number of rows and columns of the H...
PUM
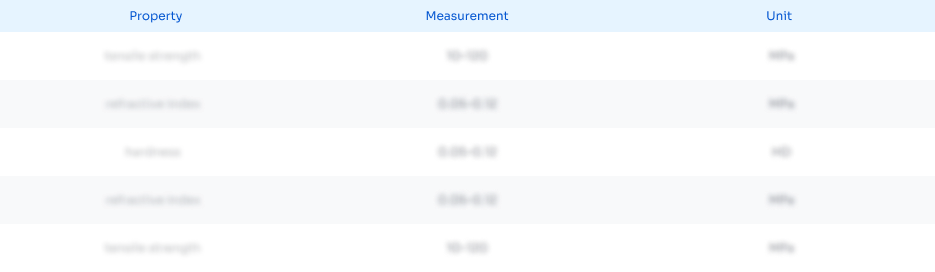
Abstract
Description
Claims
Application Information
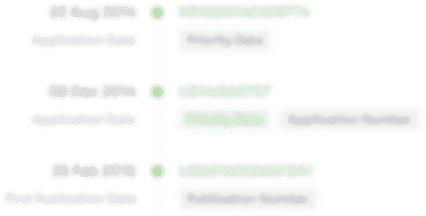
- R&D
- Intellectual Property
- Life Sciences
- Materials
- Tech Scout
- Unparalleled Data Quality
- Higher Quality Content
- 60% Fewer Hallucinations
Browse by: Latest US Patents, China's latest patents, Technical Efficacy Thesaurus, Application Domain, Technology Topic, Popular Technical Reports.
© 2025 PatSnap. All rights reserved.Legal|Privacy policy|Modern Slavery Act Transparency Statement|Sitemap|About US| Contact US: help@patsnap.com