Multi-extreme learning machine-based post-fault transient stability hierarchical evaluation method for power system
An extreme learning machine and power system technology, which is applied in the field of layered evaluation of transient stability after power system failure based on multiple extreme learning machines, can solve the problem that the transient stability evaluation cannot take into account the evaluation speed and evaluation accuracy, and achieve a comprehensive safety situation. , reasonable evaluation, and the effect of improving evaluation efficiency
- Summary
- Abstract
- Description
- Claims
- Application Information
AI Technical Summary
Problems solved by technology
Method used
Image
Examples
Embodiment
[0056] In this embodiment, the 1684-node power system is taken as the research object to determine its transient stability under different fault scenarios. The overall framework of the embodiment is as figure 1 shown. The above method mainly includes two parts: hierarchical training and hierarchical evaluation of multi-layer multiple extreme learning machines. The sample training part of each layer of multiple extreme learning machines is characterized by: power grid transient key feature extraction and centralized training of ELMs. The evaluation part of each layer of multiple extreme learning machines is characterized by: extracting key features from large data, and then sending them into a cluster of EMLs that have been trained, and finally comprehensively analyzing their outputs, giving transient stability evaluation results, and Send unconfident samples to the next layer.
[0057] As a preferred solution of the present invention, the flow chart of each layer of multipl...
PUM
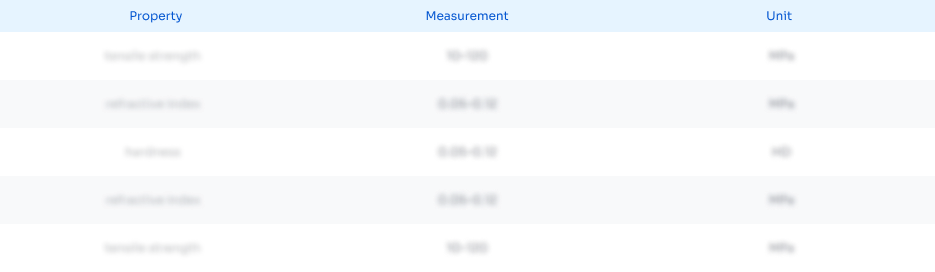
Abstract
Description
Claims
Application Information
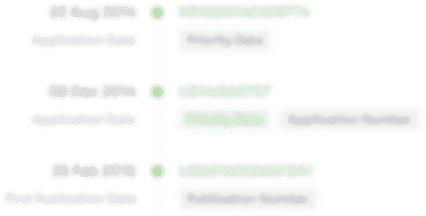
- R&D Engineer
- R&D Manager
- IP Professional
- Industry Leading Data Capabilities
- Powerful AI technology
- Patent DNA Extraction
Browse by: Latest US Patents, China's latest patents, Technical Efficacy Thesaurus, Application Domain, Technology Topic, Popular Technical Reports.
© 2024 PatSnap. All rights reserved.Legal|Privacy policy|Modern Slavery Act Transparency Statement|Sitemap|About US| Contact US: help@patsnap.com