Mean iteration-based multi-task learning model training and prediction method
A multi-task learning and model training technology, which is applied in the field of multi-task learning model training and prediction, can solve the problems of insufficient utilization of data information and low operating efficiency of multi-task learning methods
- Summary
- Abstract
- Description
- Claims
- Application Information
AI Technical Summary
Problems solved by technology
Method used
Image
Examples
Embodiment 1
[0054] A multi-task learning model training method based on mean iteration includes the following steps:
[0055] (1) Obtain sample data sets of multiple tasks, and divide the sample data sets of each task into training set and test set.
[0056] There are T tasks, and for each task t (t=1, 2, 3...T), multiple instances containing D characteristic variables are collected, and the class label corresponding to each instance of each task is obtained at the same time to obtain each task Sample data set. Among them: the class label corresponding to each instance is available {C 1 ,C 2 ,...,C k } Means that K means the total number of class labels of all instances. x 1 ,x 2 ,...,X D Represents the characteristic variables of each instance, the total number of characteristic variables is D.
[0057] The sample data set of each task is divided into training set and test set. For the sample data set of the t-th task, Train t Represents the training set corresponding to the t-th task, where...
Embodiment 2
[0095] Reference figure 1 , Is a flowchart of a prediction method based on a multi-task learning model, the method includes:
[0096] Based on the multi-task learning model training method based on mean iteration provided in Example 1, a trained multi-task learning model is obtained.
[0097] For the instance n to be predicted in the tth (t=1, 2, 3...T) task among the T tasks, obtain the D feature variables x of this instance 1,n ,x 2,n ,...,X D,n , Based on the highest priority test values of various labels on the t (t = 1, 2, 3...T) task finally output by the trained multi-task learning model And the conditional probability P of each feature variable on various labels in the tth (t=1, 2, 3...T) task t (x d |C k ), according to formula (11), the class label of the instance n can be obtained.
[0098]
Embodiment 3
[0100] A training method of student performance prediction model with multiple data sets, including
[0101] (1) There are multiple schools (one school corresponds to one task), and multiple instances with D characteristic variables are collected for each school. An example is a student. The corresponding D characteristic variables of the student can include the year of the exam, the percentage of students who are eligible for free school meals, the percentage of students at the first level of VR (the highest level of the oral reasoning test), and the gender of the school (S.GN.) , School denomination, student gender, student ethnicity, VR band (can be 1, 2 or 3). At the same time, get the class label corresponding to each instance of each task, and get the sample data set of each task. Divide the sample data set of each task t into training set and test set;
[0102] (2) Train a multi-task learning model;
[0103] (2.1) For each task, use its corresponding training set to obtain ...
PUM
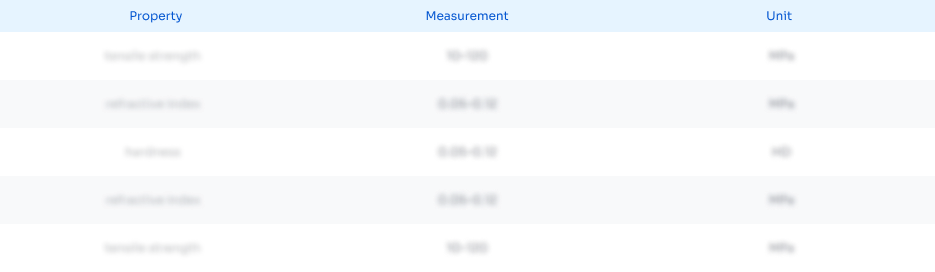
Abstract
Description
Claims
Application Information
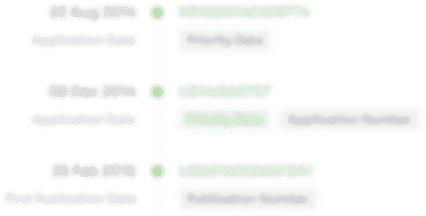
- R&D Engineer
- R&D Manager
- IP Professional
- Industry Leading Data Capabilities
- Powerful AI technology
- Patent DNA Extraction
Browse by: Latest US Patents, China's latest patents, Technical Efficacy Thesaurus, Application Domain, Technology Topic, Popular Technical Reports.
© 2024 PatSnap. All rights reserved.Legal|Privacy policy|Modern Slavery Act Transparency Statement|Sitemap|About US| Contact US: help@patsnap.com