Pedestrian detection method fusing depth perception features and kernel extreme learning machine
A kernel extreme learning machine and depth perception technology, which is applied in the field of pedestrian detection that integrates depth perception features and kernel extreme learning machines, can solve the problems of slow test speed, slow calculation speed, and cumbersome network structure, and achieves high accuracy and speed. Effect
- Summary
- Abstract
- Description
- Claims
- Application Information
AI Technical Summary
Problems solved by technology
Method used
Image
Examples
Embodiment Construction
[0035] In this example, if figure 1 As shown, the pedestrian detection algorithm of fusion depth perception features and kernel extreme learning machine applied to deep learning and kernel extreme learning machine includes the following steps:
[0036]Obtain the required training sample images from the pedestrian database, and use the preprocessed samples to construct the DAGnet convolutional neural network to complete the training; use the trained network model to extract image depth perception feature vectors for the training samples; will obtain Input the feature data of the kernel extreme learning machine classifier to complete the training; fine-tune the parameters in KELM based on K-fold cross-validation to achieve parameter optimization; preprocess the pedestrian images to be tested in the detection stage to obtain test samples, first use DAG The second-level feature map learned in the first part of the network is passed through the GVBS saliency detection algorithm to ...
PUM
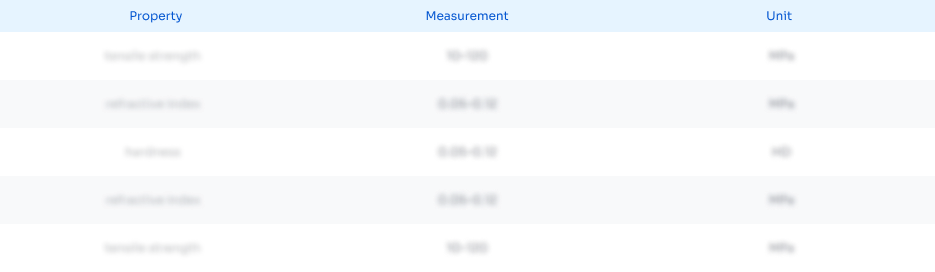
Abstract
Description
Claims
Application Information
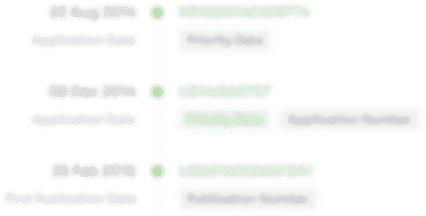
- R&D
- Intellectual Property
- Life Sciences
- Materials
- Tech Scout
- Unparalleled Data Quality
- Higher Quality Content
- 60% Fewer Hallucinations
Browse by: Latest US Patents, China's latest patents, Technical Efficacy Thesaurus, Application Domain, Technology Topic, Popular Technical Reports.
© 2025 PatSnap. All rights reserved.Legal|Privacy policy|Modern Slavery Act Transparency Statement|Sitemap|About US| Contact US: help@patsnap.com