Fault diagnosis method based on deep convolution domain adversarial transfer learning
A technology of deep convolution and transfer learning, applied in neural learning methods, testing of machine/structural components, instruments, etc., can solve the difficulties of few labeled samples, low fault diagnosis accuracy, and large number of labeled samples in rotating machinery And other issues
- Summary
- Abstract
- Description
- Claims
- Application Information
AI Technical Summary
Problems solved by technology
Method used
Image
Examples
Embodiment Construction
[0101] The specific embodiments of the present invention are described below so that those skilled in the art can understand the present invention, but it should be clear that the present invention is not limited to the scope of the specific embodiments. For those of ordinary skill in the art, as long as various changes Within the spirit and scope of the present invention defined and determined by the appended claims, these changes are obvious, and all inventions and creations using the concept of the present invention are included in the protection list.
[0102] Such as figure 1 As shown, the fault diagnosis method based on deep convolutional domain confrontation transfer learning includes the following steps:
[0103]S1. Perform segmental preprocessing on each rotating machinery sample in the auxiliary domain and the target domain to obtain corresponding preprocessing results;
[0104] S2. Input the two preprocessing results as input samples into the deep convolution domai...
PUM
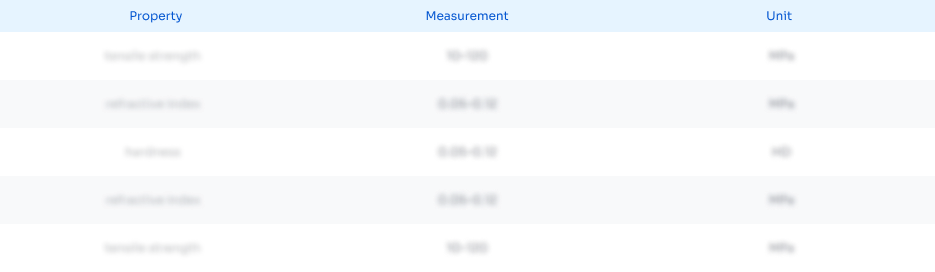
Abstract
Description
Claims
Application Information
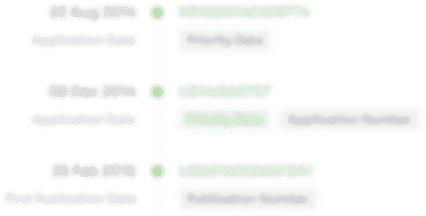
- R&D Engineer
- R&D Manager
- IP Professional
- Industry Leading Data Capabilities
- Powerful AI technology
- Patent DNA Extraction
Browse by: Latest US Patents, China's latest patents, Technical Efficacy Thesaurus, Application Domain, Technology Topic, Popular Technical Reports.
© 2024 PatSnap. All rights reserved.Legal|Privacy policy|Modern Slavery Act Transparency Statement|Sitemap|About US| Contact US: help@patsnap.com