Pulmonary nodule morphological classification method based on neural network
A technology of morphological classification and neural network, applied in biological neural network model, neural architecture, image analysis, etc., can solve the problems of limited effect of 3D CT images, loss of size information, time-consuming and labor-intensive, etc., to save manpower and material resources, Quickly detect and realize the effect of batch operations
- Summary
- Abstract
- Description
- Claims
- Application Information
AI Technical Summary
Problems solved by technology
Method used
Image
Examples
Embodiment Construction
[0056] In order to make the object, technical solution and advantages of the present invention clearer, the present invention will be further described in detail below in conjunction with the accompanying drawings.
[0057] The present invention comprises the following steps:
[0058] 1. Data preparation. This stage mainly completes the data import from the hospital data system and the calibration of the pulmonary nodules to be classified.
[0059] 2. Data preprocessing. This stage preprocesses the data, mainly including the preprocessing of the original CT image and the interception of the nodule area.
[0060] 3. Construct a multi-scale 3D residual network model, and use the collected data and calibration results to train the model.
[0061] 4. Repeat step 3 to build and train models for various morphological classifications.
[0062] 5. Use the model trained in step 3 and step 4 to classify the lung nodules automatically detected by the detection task, and output the in...
PUM
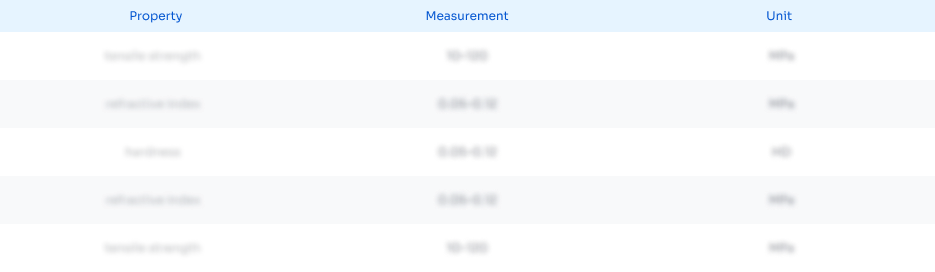
Abstract
Description
Claims
Application Information
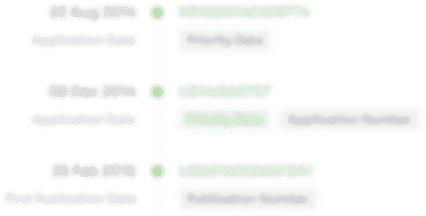
- R&D Engineer
- R&D Manager
- IP Professional
- Industry Leading Data Capabilities
- Powerful AI technology
- Patent DNA Extraction
Browse by: Latest US Patents, China's latest patents, Technical Efficacy Thesaurus, Application Domain, Technology Topic, Popular Technical Reports.
© 2024 PatSnap. All rights reserved.Legal|Privacy policy|Modern Slavery Act Transparency Statement|Sitemap|About US| Contact US: help@patsnap.com