Single cell clustering method and device, electronic equipment and storage medium
A clustering method and single-cell technology, applied in the field of bioinformatics, to achieve good prediction performance and improve clustering performance
- Summary
- Abstract
- Description
- Claims
- Application Information
AI Technical Summary
Problems solved by technology
Method used
Image
Examples
Embodiment 1
[0036] This embodiment provides a single-cell clustering method, including the following steps:
[0037] Step 1. Based on the gene expression matrix (that is, the gene expression matrix of single-cell transcriptome sequencing data, which is listed as single cell and behavioral gene expression, which can be downloaded from the public database), calculate the similarity between single-cell pairs and construct a global Feature space matrix S l ;S l The size of is N×N, N is the number of single cells, and the element S l (i, j) represents the similarity (local similarity) between single cell i and single cell j, S l Each row in represents the similarity between a single cell and all other single cells, S l Contains the global similarity information, which is the global feature space matrix;
[0038] Step 2. Based on the matrix S l , use the weighted Gaussian kernel function to calculate the similarity between single-cell pairs, and construct a sparse global similarity matrix ...
Embodiment 2
[0044] The single-cell clustering method of this embodiment, on the basis of Example 1, in the step 1, based on the gene expression matrix, calculate the Spearman correlation coefficient (Spearman correlationcoefficient) between the column vectors corresponding to two single cells ), as their similarity; the specific steps to calculate the Spearman correlation coefficient (Spearman correlation coefficient) Rs(i,j) of the column vectors corresponding to single cell i and single cell j are:
[0045] Step1: Transform the elements S(m,i) and S(m,j) corresponding to the column vectors S(:,i) and S(:,j) corresponding to single cell i and single cell j in the gene expression matrix S is the ranking (descending position) in the respective column vectors, recorded as R[S(m,i)] and R[S(m,j)], where m=1,2,...,M, M is the number of genes number, wherein the gene expression matrix S is a matrix of M rows and N columns, and N is the number of single cells;
[0046] step2: According to the ...
Embodiment 3
[0052] The single-cell clustering method of this embodiment is based on the embodiment 2, and the step 2 is specifically implemented by the following steps:
[0053] Step 2.1, based on the global feature space matrix S l , according to the value of each row element in the matrix, determine the K nearest neighbors (K nearest neighbors, KNN) of each single cell; for a single cell i, the matrix S l i line S l (i,:) divides S l The single cell corresponding to the largest K elements other than (i, i) is its K nearest neighbors, and the set of its K nearest neighbors is recorded as KNN(i); where S l (i,i) means S l The i-th element in (i,:), namely S l The elements of row i and column i; the above operation is used in the global feature space matrix S l On the basis of filtering out the weakly correlated nodes;
[0054] Step 2.2. Use the weighted Gaussian kernel function to calculate the weighted Gaussian kernel similarity D(i,j) between each single cell pair as their similar...
PUM
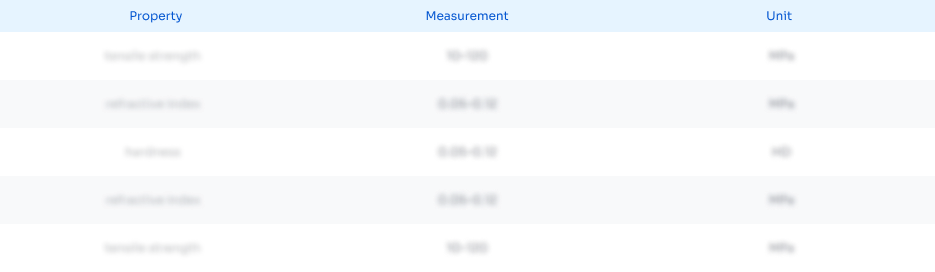
Abstract
Description
Claims
Application Information
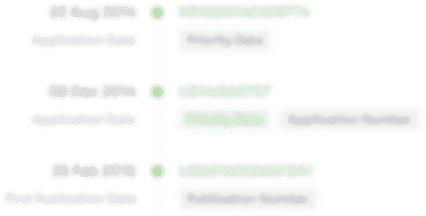
- R&D Engineer
- R&D Manager
- IP Professional
- Industry Leading Data Capabilities
- Powerful AI technology
- Patent DNA Extraction
Browse by: Latest US Patents, China's latest patents, Technical Efficacy Thesaurus, Application Domain, Technology Topic, Popular Technical Reports.
© 2024 PatSnap. All rights reserved.Legal|Privacy policy|Modern Slavery Act Transparency Statement|Sitemap|About US| Contact US: help@patsnap.com