Semi-supervised industrial image defect segmentation method based on adversarial generative network
A semi-supervised and network technology, applied in the field of computer vision, to achieve the effect of accurate defect segmentation, reduce the dependence of defect samples, and reduce the demand
- Summary
- Abstract
- Description
- Claims
- Application Information
AI Technical Summary
Problems solved by technology
Method used
Image
Examples
Embodiment Construction
[0053] The present invention will be described in detail below with reference to the accompanying drawings and preferred embodiments, and the purpose and effect of the present invention will become clearer. It should be understood that the specific embodiments described here are only used to explain the present invention and are not intended to limit the present invention.
[0054] Such as figure 1 As shown, the semi-supervised industrial image defect segmentation method based on the confrontation generation network of the present invention specifically includes the following steps:
[0055] S1: Create a negative sample library X of images containing defects respectively F and the positive sample library X of images without defects T , and pixel-label the positive and negative samples, for the positive sample x t mark y T , the default label is 1, for the negative sample x f mark y F , the pixel label at the defect of the image is 0, and the other places are 1;
[0056] ...
PUM
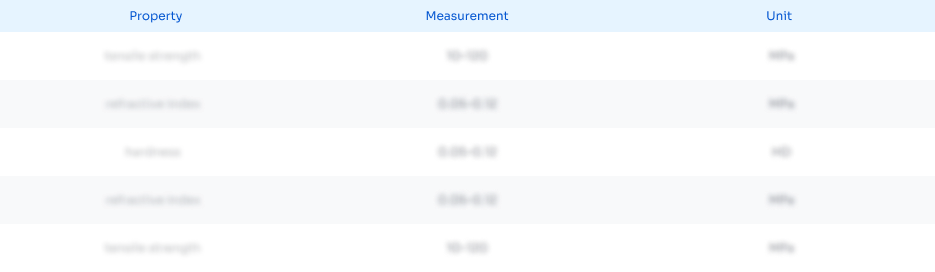
Abstract
Description
Claims
Application Information
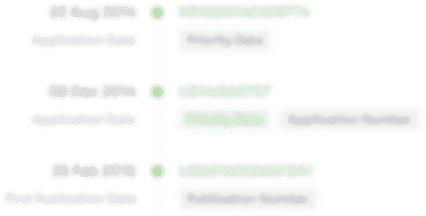
- R&D Engineer
- R&D Manager
- IP Professional
- Industry Leading Data Capabilities
- Powerful AI technology
- Patent DNA Extraction
Browse by: Latest US Patents, China's latest patents, Technical Efficacy Thesaurus, Application Domain, Technology Topic, Popular Technical Reports.
© 2024 PatSnap. All rights reserved.Legal|Privacy policy|Modern Slavery Act Transparency Statement|Sitemap|About US| Contact US: help@patsnap.com