Multi-task Triplet loss function learning method based on semantic hierarchy
A technology of loss function and learning method, applied in the field of multi-task Triplet loss function learning, can solve the problems of slow convergence of network loss function and large network influence, and achieve the effect of improving performance and improving feature separability
- Summary
- Abstract
- Description
- Claims
- Application Information
AI Technical Summary
Problems solved by technology
Method used
Image
Examples
Embodiment Construction
[0044] The present invention will be further described below in conjunction with the accompanying drawings and embodiments.
[0045] In order to make the technical means, objectives, and effects of the invention easy to understand, the present invention will be further described below in conjunction with specific embodiments.
[0046] The loss function proposed by the present invention only focuses on the metric learning part to provide more effective and separable image features, which will provide more effective applications in machine learning fields such as image recognition and retrieval.
[0047] The present invention aims at the application field of the Triplet network in the fine-grained image field, and it is found that in the development of the Triplet network, most studies only focus on category information under one semantic level. With the increase of multi-task learning requirements, it is very important to locate similar fine-grained features at different degree...
PUM
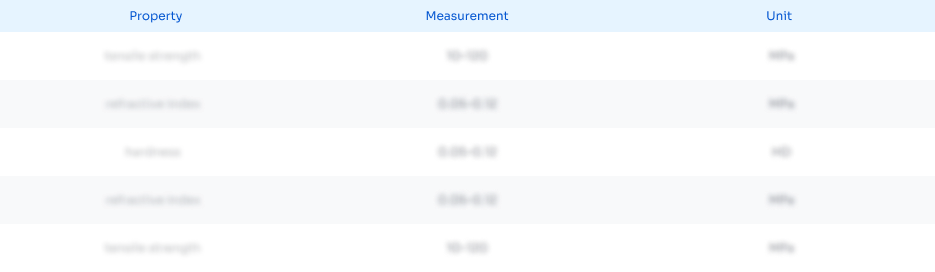
Abstract
Description
Claims
Application Information
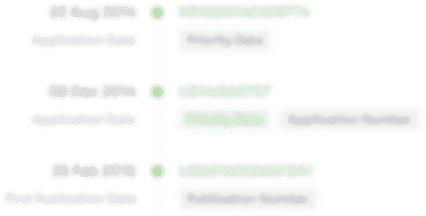
- Generate Ideas
- Intellectual Property
- Life Sciences
- Materials
- Tech Scout
- Unparalleled Data Quality
- Higher Quality Content
- 60% Fewer Hallucinations
Browse by: Latest US Patents, China's latest patents, Technical Efficacy Thesaurus, Application Domain, Technology Topic, Popular Technical Reports.
© 2025 PatSnap. All rights reserved.Legal|Privacy policy|Modern Slavery Act Transparency Statement|Sitemap|About US| Contact US: help@patsnap.com