TCN-based wind power prediction error interval evaluation method
A technology for forecasting errors and wind power, applied in forecasting, neural learning methods, instruments, etc., can solve problems such as unclear physical meaning of results, insufficient combination of wind power signal characteristics, etc., and achieve the effect of avoiding gradient disappearance
- Summary
- Abstract
- Description
- Claims
- Application Information
AI Technical Summary
Problems solved by technology
Method used
Image
Examples
Embodiment Construction
[0037] Such as Figure 1 to Figure 7 A TCN-based wind power prediction error interval evaluation method is shown, taking the actual wind power data of NREL Corporation of the United States as an example, including the following steps:
[0038] Step 1: Obtain the raw data P(t) of wind power and normalize it, and divide it into training set and test set;
[0039] Step 2: Initialize the weight of the TCN network, set the number of iterations, the number of residual modules and the number of network layers, the expansion coefficient, the size of the convolution kernel, the learning rate and the number of neurons in the hidden layer. The specific steps are as follows:
[0040] Step 2.1: Using a causal convolutional CNN model, the sequence problem can be transformed into: according to x 1 , x 2 ,...,x t to predict y 1 ,y 2 ,...,y t , the definition of causal convolution, filter F=(f1 , f 2 ,...,f k ), sequence X=(x 1 , x 2 ,...,x T ), at x t The causal convolution at is:...
PUM
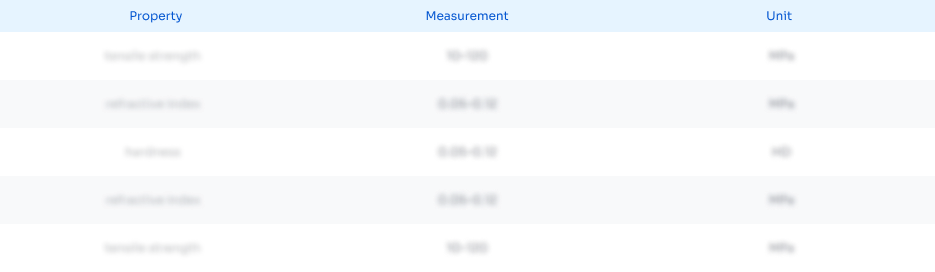
Abstract
Description
Claims
Application Information
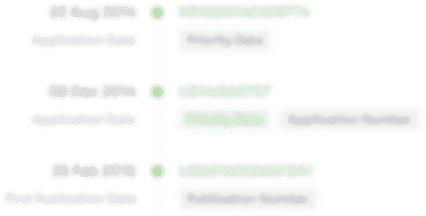
- R&D
- Intellectual Property
- Life Sciences
- Materials
- Tech Scout
- Unparalleled Data Quality
- Higher Quality Content
- 60% Fewer Hallucinations
Browse by: Latest US Patents, China's latest patents, Technical Efficacy Thesaurus, Application Domain, Technology Topic, Popular Technical Reports.
© 2025 PatSnap. All rights reserved.Legal|Privacy policy|Modern Slavery Act Transparency Statement|Sitemap|About US| Contact US: help@patsnap.com