Multi-scale residual attention network image super-resolution reconstruction method based on attention
A super-resolution reconstruction, network image technology, applied in the field of multi-scale residual attention network image super-resolution reconstruction based on attention, can solve the problems of increased computational burden, waste of limited network computing resources, loss of high-frequency information, etc. To achieve the effect of reducing the reconstruction error
- Summary
- Abstract
- Description
- Claims
- Application Information
AI Technical Summary
Problems solved by technology
Method used
Image
Examples
Embodiment
[0050] Such as figure 1 As shown, the embodiment of the present invention provides an attention-based multi-scale residual attention network image super-resolution reconstruction method, comprising the following steps:
[0051] Step S1: select the public image data set as the image set to be tested, and divide the image set to be tested into an image training set and an image test set according to a certain ratio, and perform image preprocessing operations.
[0052] In the specific implementation process, the public image data set is selected as the image set to be tested, and the image set to be tested is divided into an image training set and an image test set according to a certain ratio, and the method for performing image preprocessing operations includes:
[0053] First, the DIV2K dataset is used as the experimental image set, N images are randomly selected from multiple high-resolution images as the experimental training set, and the remaining M images are used as the e...
PUM
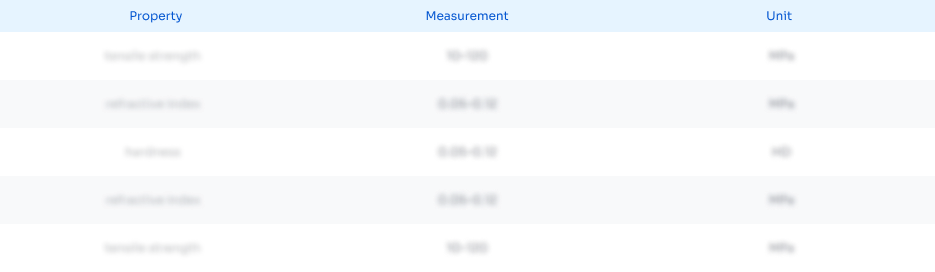
Abstract
Description
Claims
Application Information
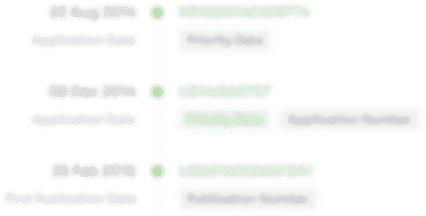
- R&D Engineer
- R&D Manager
- IP Professional
- Industry Leading Data Capabilities
- Powerful AI technology
- Patent DNA Extraction
Browse by: Latest US Patents, China's latest patents, Technical Efficacy Thesaurus, Application Domain, Technology Topic, Popular Technical Reports.
© 2024 PatSnap. All rights reserved.Legal|Privacy policy|Modern Slavery Act Transparency Statement|Sitemap|About US| Contact US: help@patsnap.com