Coupling analysis method of multi-channel electroencephalogram based on multi-scale multi-variate transfer entropy
A coupling analysis and multivariate technology, applied in sensors, diagnostic recording/measurement, medical science, etc., to achieve considerable social and economic benefits
- Summary
- Abstract
- Description
- Claims
- Application Information
AI Technical Summary
Problems solved by technology
Method used
Image
Examples
specific Embodiment approach
[0033] Such as figure 1 As shown, the method steps are as follows:
[0034] Step 1. Use a 32-lead Neuracle device to collect multi-channel EEG signals.
[0035] EEG signal collection: EEG electrodes adopt the international standard 10-20 electrode placement standard. Multi-channel electrical signal synchronous acquisition experiment was carried out under 20% static grip output movement of the hand. From the 32-lead Neuracle EEG acquisition device, FC3, FC4, C3, CZ, C4, CP3, CP4 7 channels of EEG data were recorded corresponding to the exercise EEG signal, and then the connectivity of the cortical sensorimotor area was analyzed.
[0036] Step 2: Remove baseline drift, EMG interference, eye movement interference and 50Hz power frequency interference on the collected EEG signals based on matlab data analysis software;
[0037] Step 3. Use the coarse-grained analysis method to perform scaled analysis on the selected 7-channel EEG signals, and construct X={x based on the multi-channel EE...
PUM
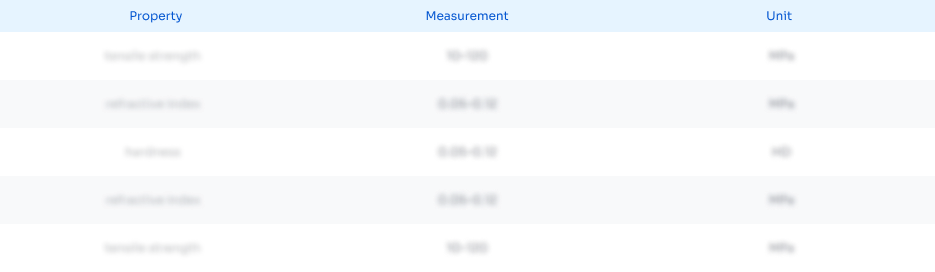
Abstract
Description
Claims
Application Information
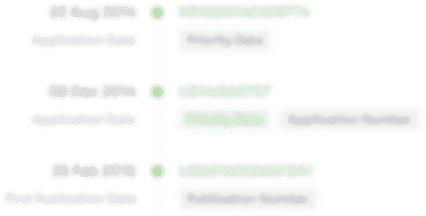
- R&D
- Intellectual Property
- Life Sciences
- Materials
- Tech Scout
- Unparalleled Data Quality
- Higher Quality Content
- 60% Fewer Hallucinations
Browse by: Latest US Patents, China's latest patents, Technical Efficacy Thesaurus, Application Domain, Technology Topic, Popular Technical Reports.
© 2025 PatSnap. All rights reserved.Legal|Privacy policy|Modern Slavery Act Transparency Statement|Sitemap|About US| Contact US: help@patsnap.com