Rail fastener defect recognition algorithm based on deep learning
A track fastener and defect identification technology, which is applied in the field of track fastener defect identification algorithm, can solve problems such as uneven illumination, poor separability, and influence on feature extraction, and achieve improved accuracy and stability, strong feature expression, and Guaranteed the effect of accuracy
- Summary
- Abstract
- Description
- Claims
- Application Information
AI Technical Summary
Problems solved by technology
Method used
Image
Examples
Embodiment Construction
[0057] The technical solution of the present invention will be described in further detail below in conjunction with the accompanying drawings and embodiments.
[0058] 1 Three-dimensional laser imaging technology and fastener detection system
[0059] 1.1 Fastener detection system
[0060] In the previous research, the research group independently developed and designed a rail inspection vehicle for carrying the inspection system. Based on the existing multi-functional inspection system research foundation, the fastener inspection system was developed. In the first-generation detection system, only one set of laser-camera acquisition unit is used to collect railway track data, and the laser is the green line laser at this time. However, the green laser consumes a lot of energy and will generate a lot of heat, so a heat dissipation system must be installed in the collection box, so the space occupied by the collection box is relatively large. In the development of the second...
PUM
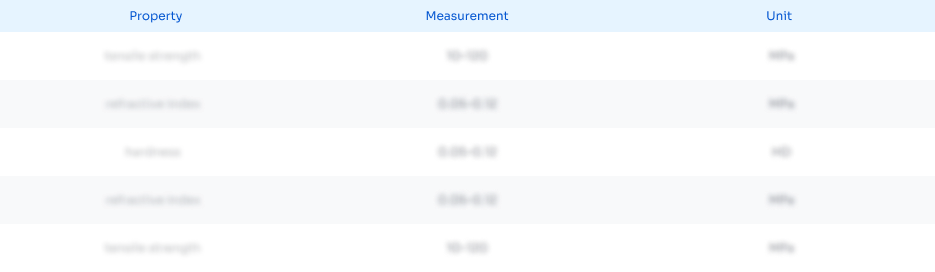
Abstract
Description
Claims
Application Information
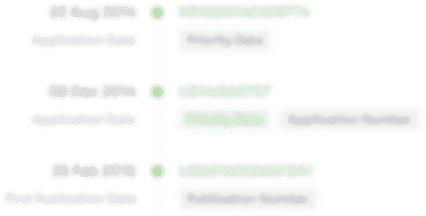
- R&D
- Intellectual Property
- Life Sciences
- Materials
- Tech Scout
- Unparalleled Data Quality
- Higher Quality Content
- 60% Fewer Hallucinations
Browse by: Latest US Patents, China's latest patents, Technical Efficacy Thesaurus, Application Domain, Technology Topic, Popular Technical Reports.
© 2025 PatSnap. All rights reserved.Legal|Privacy policy|Modern Slavery Act Transparency Statement|Sitemap|About US| Contact US: help@patsnap.com