Malicious code detection method and system
A malicious code detection and malicious code technology, applied in the direction of instruments, calculations, electrical digital data processing, etc., can solve the problems of fuzzy category boundaries, missing data part features, no obvious improvement, etc.
- Summary
- Abstract
- Description
- Claims
- Application Information
AI Technical Summary
Problems solved by technology
Method used
Image
Examples
Embodiment
[0121] In this embodiment, a malicious code detection method is designed. A malicious code detection model based on feature integration and data partitioning is designed. The model is mainly divided into two parts. The first part uses TF-IDF (Term Frequency-Inverse Document Frequency, term frequency-inverse text frequency ) and the Doc2vec algorithm to extract the features of the action sequence of the malicious code. The second part is based on the first part, and uses the clustering-based ensemble classification improvement model to classify the malicious code. Such as figure 1 As shown, the specific content of this embodiment is as follows:
[0122] S1, TF-IDF and Doc2vec extract malicious code family feature fusion
[0123] Treat the Windows API action sequence in the running process of each malicious code as a contextual text, and use TF-IDF and Doc2vec for feature extraction;
[0124] TF-IDF is a statistical method used to evaluate the importance of specific words in t...
PUM
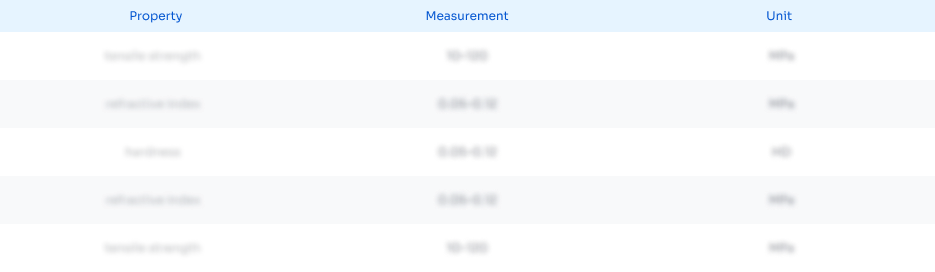
Abstract
Description
Claims
Application Information
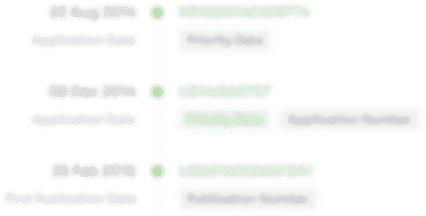
- R&D
- Intellectual Property
- Life Sciences
- Materials
- Tech Scout
- Unparalleled Data Quality
- Higher Quality Content
- 60% Fewer Hallucinations
Browse by: Latest US Patents, China's latest patents, Technical Efficacy Thesaurus, Application Domain, Technology Topic, Popular Technical Reports.
© 2025 PatSnap. All rights reserved.Legal|Privacy policy|Modern Slavery Act Transparency Statement|Sitemap|About US| Contact US: help@patsnap.com