Modulated signal time-frequency diagram classification system based on generative adversarial network and operation method thereof
A technology for modulating signals and classification systems, applied in biological neural network models, image analysis, neural learning methods, etc., can solve the problems of insufficient training signal classification neural network data sets, inability to dig deep into signal features, etc., to improve accuracy and the effect of robustness
- Summary
- Abstract
- Description
- Claims
- Application Information
AI Technical Summary
Problems solved by technology
Method used
Image
Examples
Embodiment 1
[0071] A modulated signal time-frequency graph classification system based on GAN. It is a specific application of GAN model in modulation signal classification. It is composed of GAN model and auxiliary classifier model, including IQ signal time-frequency graph Transformation module, generator module, discriminator module;
[0072] The IQ signal time-frequency diagram conversion module and the generator module are all connected to the discriminator module; the discriminator module includes an auxiliary classifier module;
[0073] The IQ signal time-frequency diagram transformation module is used to convert the IQ two-way original modulation signal into a time-frequency diagram form through short-time Fourier transform; the IQ signal time-frequency diagram transformation module runs before generating the confrontational network architecture to generate the confrontational network architecture Including the generator module and the discriminator module; transforming the IQ two-...
Embodiment 2
[0079] According to a kind of modulation signal time-frequency diagram classification system based on generating confrontation network described in embodiment 1, its difference is:
[0080] The IQ signal time-frequency map transformation module includes four parameters for adjustment, including: short-time Fourier transform window type win_cls, short-time Fourier transform window length win_sz, short-time Fourier transform point number nfft, and overlapping point number noverlap. The short-time Fourier transform window type win_cls determines the effect of a short-time Fourier transform, and the corresponding window type can be selected according to different needs. The short-time Fourier transform window type win_cls is a Hanning window, and the window length is and the number of short-time Fourier transform points determines the accuracy of the time-frequency domain. The short-time Fourier transform window length win_sz is 40 signal points, the number of short-time Fourier tr...
Embodiment 3
[0088] The operating method of a modulation signal time-frequency graph classification system based on generating an adversarial network described in Embodiment 2, such as image 3 shown, including the following steps:
[0089] (1) Data preprocessing
[0090] The data set preprocessing is the first step of the entire modulation signal time-frequency diagram classification system. The IQ two-way original modulation signal passes through the IQ signal time-frequency diagram conversion module to convert the IQ two-way original modulation signal into the form of a time-frequency diagram; In order to better explore the deep-level features of the signal, in the IQ signal time-frequency map transformation module, set the short-time Fourier transform window length win_sz to 40 signal points, and set the step to 38 signal points, each time Step by 2 signal points. Such a setting method can better mine the deep-level features of the signal, and provide a better classification basis fo...
PUM
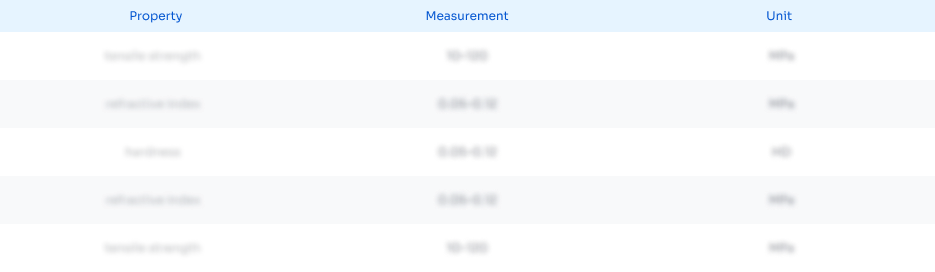
Abstract
Description
Claims
Application Information
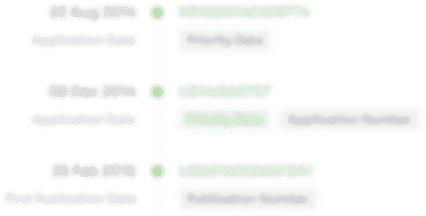
- R&D Engineer
- R&D Manager
- IP Professional
- Industry Leading Data Capabilities
- Powerful AI technology
- Patent DNA Extraction
Browse by: Latest US Patents, China's latest patents, Technical Efficacy Thesaurus, Application Domain, Technology Topic.
© 2024 PatSnap. All rights reserved.Legal|Privacy policy|Modern Slavery Act Transparency Statement|Sitemap