Neural network model pruning method and system based on adaptive batch standardization
A neural network model and adaptive technology, applied in the field of neural network model pruning, can solve the problem of large time consumption, achieve the advantage of accuracy and avoid huge time consumption.
- Summary
- Abstract
- Description
- Claims
- Application Information
AI Technical Summary
Problems solved by technology
Method used
Image
Examples
Embodiment 1
[0027] A neural network model pruning method based on adaptive batch normalization provided by an embodiment of the present invention, such as figure 2 shown, including the following steps:
[0028] Step S1: Aiming at an L-layer neural network model, randomly sample L floating-point numbers in [0,R] (01 ,r 2 ,...,r L ) as a pruning strategy.
[0029] In the embodiment of the present invention, a convolutional layer with a size of 3×3 of all convolution kernels of MobileNetV1 is used as a prunable layer (L=14), and 14 [0, R] (01 ,r 2 ,...,r L ) as a pruning strategy, each element r in the vector 1 Represents the proportion of the convolution kernel that needs to be reduced in layer l, that is, the pruning rate of each layer. The pruning strategy is reserved to meet the preset computing resource restrictions, including the preset calculation operation limit, the preset parameter limit, the preset At least one of the calculation delay restrictions, for example, the number o...
Embodiment 2
[0049] Embodiments of the present invention provide a neural network model pruning system based on adaptive batch normalization, such as Figure 4 shown, including the following steps:
[0050] The pruning strategy generation module 1 is used to randomly sample L floating-point numbers in [0, R] (01 ,r 2 ,...,r L ) as a pruning strategy; this module executes the method described in step S1 in Embodiment 1, which will not be repeated here.
[0051] The pruning model candidate set generation module 2 is used to perform pruning based on the pruning strategy neural network model respectively, and generate a pruning model candidate set composed of the pruned model; this module executes the description of step S2 in Embodiment 1 method, which will not be repeated here.
[0052] The statistical parameter update module of the batch normalization layer is used to update the statistical parameters of the batch normalization layer by using the adaptive batch normalization method respe...
Embodiment 3
[0056] An embodiment of the present invention provides a computer device, such as Figure 5 As shown, it includes: at least one processor 401 , such as a CPU (Central Processing Unit, central processing unit), at least one communication interface 403 , memory 404 , and at least one communication bus 402 . Wherein, the communication bus 402 is used to realize connection and communication between these components. Wherein, the communication interface 403 may include a display screen (Display) and a keyboard (Keyboard), and the optional communication interface 403 may also include a standard wired interface and a wireless interface. The memory 404 may be a high-speed RAM memory (Ramdom Access Memory, volatile random access memory), or a non-volatile memory (non-volatile memory), such as at least one disk memory. Optionally, the memory 404 may also be at least one storage device located away from the aforementioned processor 401 . The processor 401 may execute the neural network...
PUM
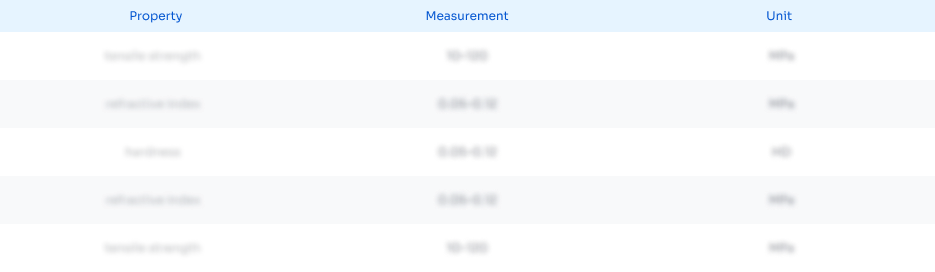
Abstract
Description
Claims
Application Information
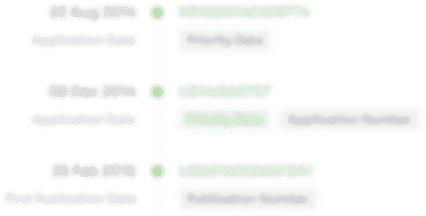
- R&D Engineer
- R&D Manager
- IP Professional
- Industry Leading Data Capabilities
- Powerful AI technology
- Patent DNA Extraction
Browse by: Latest US Patents, China's latest patents, Technical Efficacy Thesaurus, Application Domain, Technology Topic, Popular Technical Reports.
© 2024 PatSnap. All rights reserved.Legal|Privacy policy|Modern Slavery Act Transparency Statement|Sitemap|About US| Contact US: help@patsnap.com