Generative adversarial network-based adversarial attack sample generation method
A network and sample technology, applied in the field of adversarial attack sample generation, can solve problems such as low robustness, lack of learning ability in data distribution, etc.
- Summary
- Abstract
- Description
- Claims
- Application Information
AI Technical Summary
Problems solved by technology
Method used
Image
Examples
Embodiment 1
[0109] figure 1 A flowchart representing a method for generating an adversarial attack sample based on a generative adversarial network, figure 2 Denotes the first training framework based on Generator Adversarial Networks, including the generator G 1 , generator G 2 , discriminator D 1 and target attack network F.
[0110] Among them, in this embodiment, the generator G 1 Use ResNet's basic residual module as a deconvolutional neural network to upsample the tensor, random noise z and random condition vector c fake as generator G 1 The input of is obtained by deconvolution network up-sampling to obtain a fake sample image x fake ; generator G 2 Use ResNet's basic residual module as a deconvolutional neural network to upsample tensors, and random noise z as a generator G 2 The input of the deconvolution network is up-sampled to obtain the anti-disturbance x pb ; The target attack network F uses VGG as the network structure to counter the attack sample x adv As the in...
Embodiment 2
[0133] Figure 8 Denotes the second training framework based on the generator confrontation network, including the generator G 1 , generator G 2 , discriminator D 1 and the discriminator D 2 The target attacks network F.
[0134] Among them, in this embodiment, the generator G 1 Use ResNet's basic residual module as a deconvolutional neural network to upsample the tensor, random noise z and random condition vector c fake as generator G 1 The input of is obtained by deconvolution network up-sampling to obtain a fake sample image x fake ; generator G 2 Use ResNet's basic residual module as a deconvolutional neural network to upsample tensors, and random noise z as a generator G 2 The input of the deconvolution network is up-sampled to obtain the anti-disturbance x pb ; The target attack network F uses VGG as the network structure to counter the attack sample x adv As the input of the target attack network F, the output confrontation loss; the discriminator D 1 Using R...
PUM
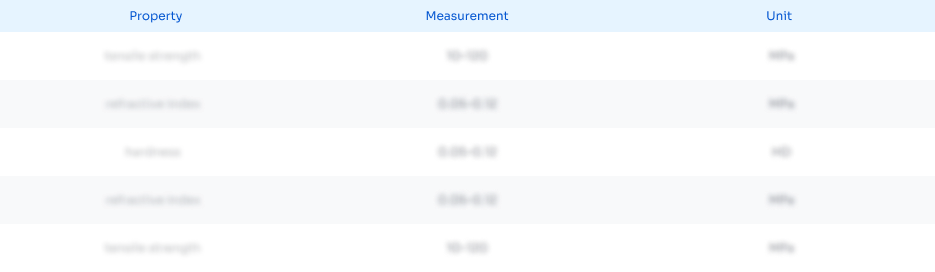
Abstract
Description
Claims
Application Information
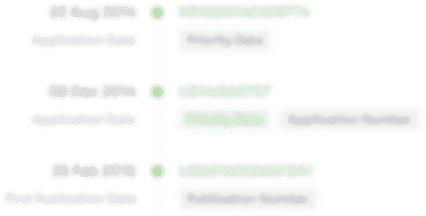
- R&D Engineer
- R&D Manager
- IP Professional
- Industry Leading Data Capabilities
- Powerful AI technology
- Patent DNA Extraction
Browse by: Latest US Patents, China's latest patents, Technical Efficacy Thesaurus, Application Domain, Technology Topic.
© 2024 PatSnap. All rights reserved.Legal|Privacy policy|Modern Slavery Act Transparency Statement|Sitemap