LSTM neural network cyclic hydrological forecasting method based on mutual information
A neural network and hydrological forecasting technology, applied in the field of data processing, can solve the problems of independent input and output of difficult physical relationships, slow convergence speed, overfitting, etc., to achieve automatic capture of effective features, improved forecasting accuracy, and high forecasting accuracy. Effect
- Summary
- Abstract
- Description
- Claims
- Application Information
AI Technical Summary
Problems solved by technology
Method used
Image
Examples
Embodiment Construction
[0055] In order to make the object, technical solution and advantages of the present invention clearer, the present invention will be further described in detail below in conjunction with the examples. It should be understood that the specific embodiments described here are only used to explain the present invention, not to limit the present invention.
[0056] For the problems existing in the prior art, the present invention provides a kind of LSTM neural network cycle hydrological prediction method based on mutual information, below in conjunction with accompanying drawing the present invention is described in detail.
[0057] Such as figure 1 As shown, the mutual information-based LSTM neural network cycle hydrological prediction method provided by the embodiment of the present invention comprises the following steps:
[0058] S101: Screen and classify the original data through mutual information analysis, and use hydrological characteristics such as rainfall, reservoir wa...
PUM
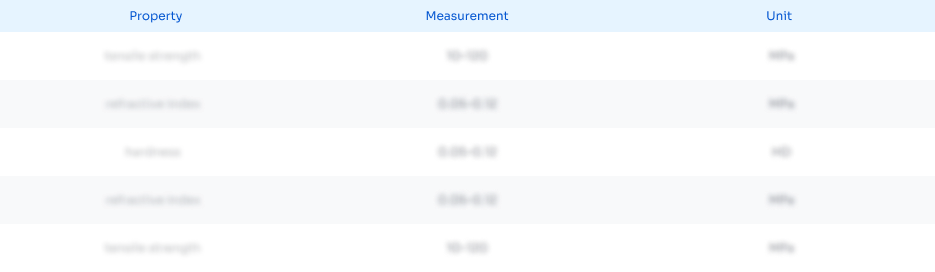
Abstract
Description
Claims
Application Information
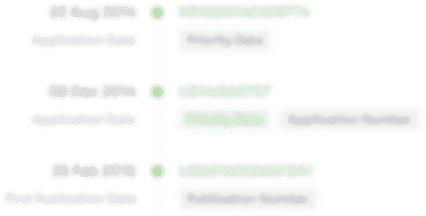
- R&D Engineer
- R&D Manager
- IP Professional
- Industry Leading Data Capabilities
- Powerful AI technology
- Patent DNA Extraction
Browse by: Latest US Patents, China's latest patents, Technical Efficacy Thesaurus, Application Domain, Technology Topic, Popular Technical Reports.
© 2024 PatSnap. All rights reserved.Legal|Privacy policy|Modern Slavery Act Transparency Statement|Sitemap|About US| Contact US: help@patsnap.com