Porosity prediction method based on multi-layer long short-term memory neural network model
A neural network model, long-term and short-term memory technology, applied in biological neural network models, neural learning methods, neural architectures, etc., can solve problems such as difficulty in ensuring authenticity, low accuracy, and difficulty in effectively predicting sequence data, etc., to achieve a solution Effect of Gradient Diffusion vs. Gradient Explosion, Good Predictive Performance
- Summary
- Abstract
- Description
- Claims
- Application Information
AI Technical Summary
Problems solved by technology
Method used
Image
Examples
Embodiment Construction
[0043]The present invention will be described in detail below in conjunction with the accompanying drawings and specific embodiments. This embodiment is carried out on the premise of the technical solution of the present invention, and detailed implementation and specific operation process are given, but the protection scope of the present invention is not limited to the following embodiments.
[0044] Such as figure 1 As shown, there are four interactive layers with special connections in the LSTM provided by the present invention, and the concept of "gate" is introduced in the LSTM to control the mutual transfer calculation in the hidden unit. The unit state C is the key to the processing of the hidden unit, and the previous information memorized in C runs through the calculation and processing of the steps before and after the LSTM. The input of the current moment and the state of the previous hidden unit are processed through the three gate structures of the interactive l...
PUM
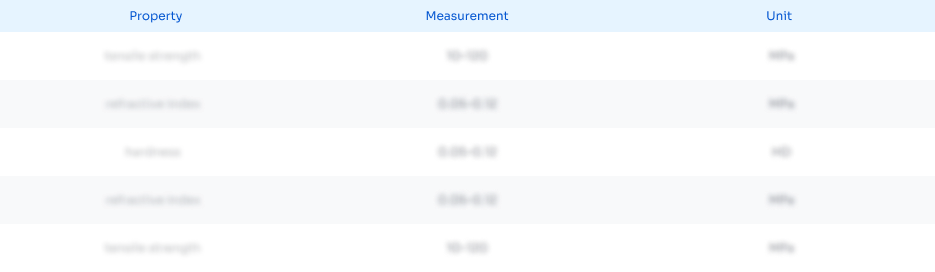
Abstract
Description
Claims
Application Information
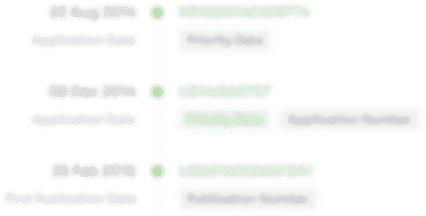
- R&D Engineer
- R&D Manager
- IP Professional
- Industry Leading Data Capabilities
- Powerful AI technology
- Patent DNA Extraction
Browse by: Latest US Patents, China's latest patents, Technical Efficacy Thesaurus, Application Domain, Technology Topic, Popular Technical Reports.
© 2024 PatSnap. All rights reserved.Legal|Privacy policy|Modern Slavery Act Transparency Statement|Sitemap|About US| Contact US: help@patsnap.com