SAR image change detection method based on multi-region convolutional neural network
A convolutional neural network and image change detection technology, which is applied in the directions of instruments, character and pattern recognition, scene recognition, etc., can solve unsupervised methods with poor noise robustness and adaptability, influence of model generalization ability, and difficulty in obtaining change information and other problems to achieve the effect of alleviating the model over-fitting problem
- Summary
- Abstract
- Description
- Claims
- Application Information
AI Technical Summary
Problems solved by technology
Method used
Image
Examples
Embodiment Construction
[0074] In the following description, various aspects of the present invention will be described. However, those skilled in the art can implement the present invention by using only some or all of the structures or processes of the present invention. For clarity of explanation, specific numbers, arrangements and sequences are set forth, but it will be apparent that the invention may be practiced without these specific details. In other instances, well-known features have not been described in detail in order not to obscure the invention.
[0075] refer to figure 1 Concrete steps that the present invention realizes:
[0076] Step 1: Perform difference analysis on two multi-temporal SAR images of the same location to obtain a difference image:
[0077] The difference analysis is carried out using the log ratio to the two multi-temporal SAR images, and the differential image of the multi-temporal SAR images is obtained;
[0078] The calculation process of the difference image ...
PUM
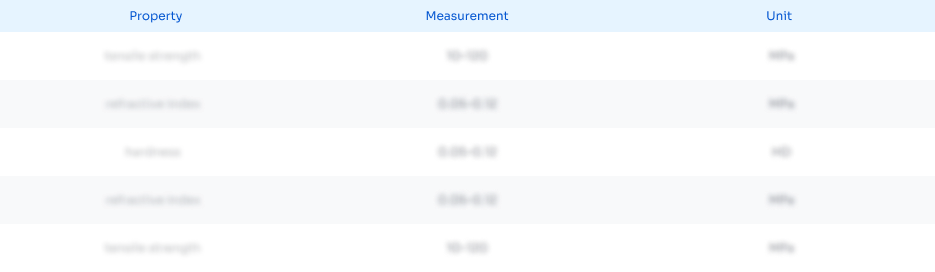
Abstract
Description
Claims
Application Information
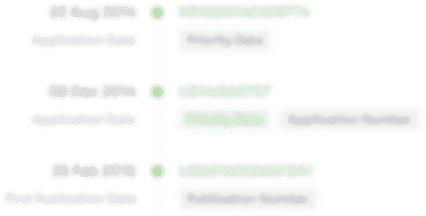
- R&D Engineer
- R&D Manager
- IP Professional
- Industry Leading Data Capabilities
- Powerful AI technology
- Patent DNA Extraction
Browse by: Latest US Patents, China's latest patents, Technical Efficacy Thesaurus, Application Domain, Technology Topic, Popular Technical Reports.
© 2024 PatSnap. All rights reserved.Legal|Privacy policy|Modern Slavery Act Transparency Statement|Sitemap|About US| Contact US: help@patsnap.com