Text-to-image generation method of adaptive attribute and instance mask embedded graph
An image generation and self-adaptive technology, applied in 2D image generation, image coding, image data processing, etc., can solve problems such as pixel overlap and occlusion, inability to synthesize high-resolution images, and lack of instance shape mask constraints
- Summary
- Abstract
- Description
- Claims
- Application Information
AI Technical Summary
Problems solved by technology
Method used
Image
Examples
Embodiment Construction
[0058] In order to make the object, technical solution and advantages of the present invention clearer, the present invention will be further described in detail below in conjunction with the accompanying drawings.
[0059] Such as figure 1 As shown, in an embodiment of the present invention, a text-to-image generation method of an adaptive attribute and an instance mask embedding graph is provided, and the method includes the following steps:
[0060] Step S1, according to the input text, use the frame regression network of the preset encoder-decoder structure to obtain the position and label information of the instance bounding box corresponding to each word in the text, and integrate the bounding boxes generated by all instances The location and label information of , get 64×64, 128×128 and 256×256 semantic layout;
[0061] The specific process is as figure 2 As shown, first use the pre-trained Bi-LSTM as a text encoder to encode the text into word vectors and a sentence...
PUM
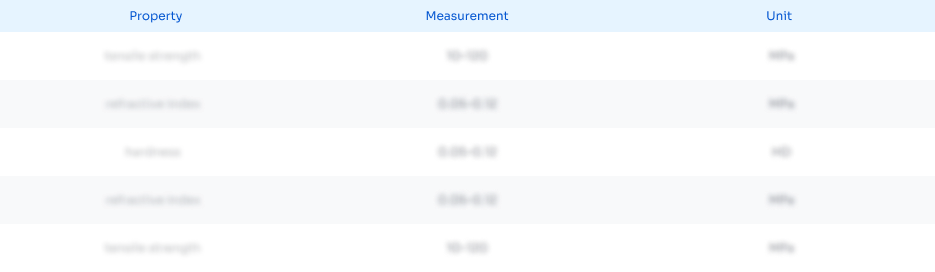
Abstract
Description
Claims
Application Information
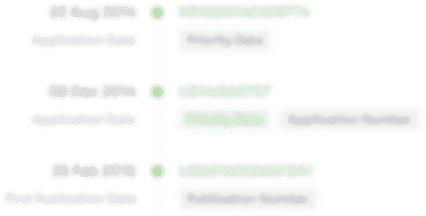
- R&D Engineer
- R&D Manager
- IP Professional
- Industry Leading Data Capabilities
- Powerful AI technology
- Patent DNA Extraction
Browse by: Latest US Patents, China's latest patents, Technical Efficacy Thesaurus, Application Domain, Technology Topic, Popular Technical Reports.
© 2024 PatSnap. All rights reserved.Legal|Privacy policy|Modern Slavery Act Transparency Statement|Sitemap|About US| Contact US: help@patsnap.com