Baseline-based image super-resolution reconstruction method and system
A super-resolution reconstruction and high-resolution image technology, applied in the field of computer vision, can solve the problems of no original image, blurred image, etc.
- Summary
- Abstract
- Description
- Claims
- Application Information
AI Technical Summary
Problems solved by technology
Method used
Image
Examples
Embodiment 1
[0041] In one or more embodiments, a baseline-based image super-resolution reconstruction method is disclosed, referring to Fig. 1(a)-(b), wherein Fig. 1(b) is a generator model; including the following steps:
[0042] Step 1: Select the training set;
[0043] A data set composed of 91 images was selected as a training sample, and the data set was divided into 24,800 sub-images, each of which was 33X33 in size, and trained multiple times to prove the baseline-based super-resolution reconstruction model proposed by the embodiment of the present invention availability.
[0044] Step 2: Build a baseline-based super-resolution reconstruction model. refer to Figure 2-Figure 3 ,in, figure 2 For the SRCNN model, image 3 as the baseline block diagram model.
[0045] Baseline-based convolutional neural network models input low-resolution images and output high-resolution images.
[0046] Two continuous residual network models built with the SRCNN network as the baseline. Each ...
Embodiment 2
[0072] In one or more embodiments, a baseline-based image super-resolution reconstruction system is disclosed, comprising:
[0073] means for building baseline-based convolutional neural network models;
[0074] A device for constructing an image super-resolution reconstruction model based on a conditional generative confrontation network;
[0075] It is used to input the low-resolution image to be reconstructed into the baseline-based convolutional neural network model, and the output of the neural network model is used as the input of the image super-resolution reconstruction model based on the conditional generation confrontation network, and finally obtain the super-resolution reconstruction image device.
[0076] The specific implementation method of the above device is the same as the method disclosed in the first embodiment, and will not be repeated here.
Embodiment 3
[0078] In one or more embodiments, a terminal device is disclosed, including a server, the server includes a memory, a processor, and a computer program stored on the memory and operable on the processor, and the processor executes the The program implements the baseline-based image super-resolution reconstruction method in Embodiment 1. For the sake of brevity, details are not repeated here.
PUM
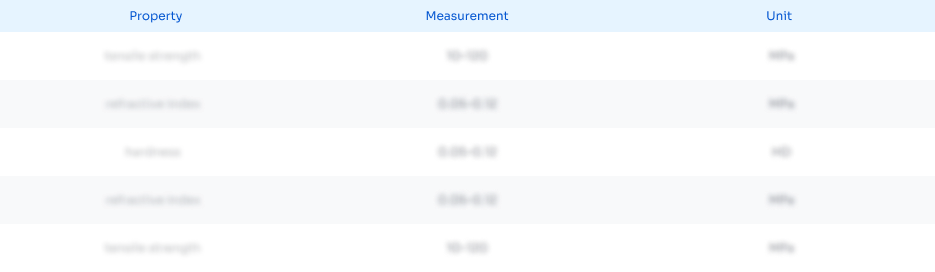
Abstract
Description
Claims
Application Information
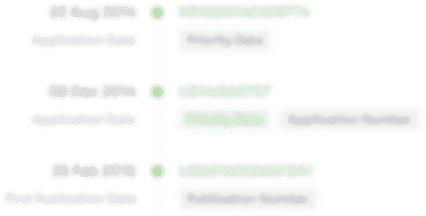
- R&D Engineer
- R&D Manager
- IP Professional
- Industry Leading Data Capabilities
- Powerful AI technology
- Patent DNA Extraction
Browse by: Latest US Patents, China's latest patents, Technical Efficacy Thesaurus, Application Domain, Technology Topic, Popular Technical Reports.
© 2024 PatSnap. All rights reserved.Legal|Privacy policy|Modern Slavery Act Transparency Statement|Sitemap|About US| Contact US: help@patsnap.com