Federated learning method and system based on batch size and gradient compression ratio adjustment
A learning method and compression rate technology, applied in neural learning methods, neural architecture, biological neural network models, etc., can solve problems such as the influence of model convergence rate, and achieve the effect of ensuring training accuracy, improving convergence rate, and reducing pressure.
- Summary
- Abstract
- Description
- Claims
- Application Information
AI Technical Summary
Problems solved by technology
Method used
Image
Examples
Embodiment 1
[0068] The federated learning method based on adjusting the batch size and gradient compression rate provided by this embodiment is applicable to the scenario where multiple mobile terminals and an edge server connected to a communication hotspot (such as a base station) jointly train an artificial intelligence model. For other wireless communication technologies, They can work in the same working mode, so in this embodiment, the situation of mobile communication technology is mainly considered.
[0069] In this embodiment, each terminal uses a batch method to perform local calculations, and the gradient compression method is quantization. In particular, the quantization adopts fixed-length quantization, and the quantization process is as follows: figure 2 , the gradient information is quantized and encoded by a certain bit, and then transmitted to the edge server as gradient information. When a high quantization bit is used to represent the gradient, the original gradient in...
Embodiment 2
[0097] The adjustment method provided in this embodiment is applicable to the scene where multiple mobile terminals and an edge server connected to a communication hotspot (such as a base station) jointly train an artificial intelligence model. For other wireless communication technologies, they can work in the same working mode, so in In this embodiment, the case of mobile communication is mainly considered.
[0098] In this embodiment, each terminal uses a batch method to perform local calculations, and the gradient compression method is thinning. In particular, the thinning method is to select some relatively large gradients for transmission. The thinning process is as follows: Figure 5 , after the gradient information is sparse, the selected gradient information and its number are transmitted to the edge server. When more gradient information is retained, the loss of gradient information can be reduced, and the amount of transmitted information is also increased; when less ...
Embodiment 3
[0127] Embodiment 3 provides a federated learning system based on adjusting batch size and gradient compression rate, including an edge server connected to the base communication terminal, and multiple terminals wirelessly communicating with the edge server,
[0128] The edge server adjusts the batch size and gradient compression rate of the terminal according to the current batch size and gradient compression rate in combination with the computing power of the terminal and the communication capability between the edge server and the terminal, and calculates the adjusted batch size and gradient compression rate transmitted to the terminal;
[0129] The terminal performs model learning according to the received batch size, and outputs the gradient information obtained by model learning to the edge server after being compressed according to the received extraction compression rate;
[0130] After the edge server averages all the received gradient information, it synchronizes the...
PUM
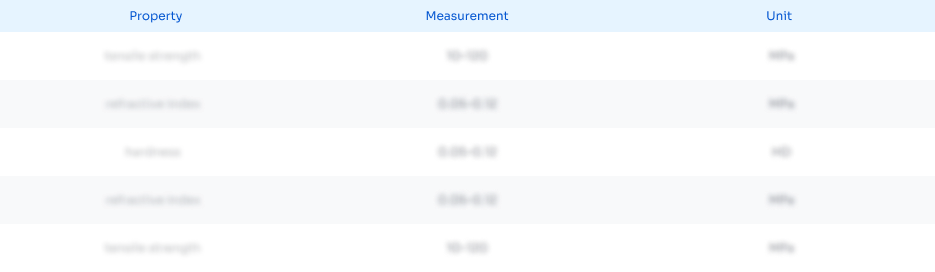
Abstract
Description
Claims
Application Information
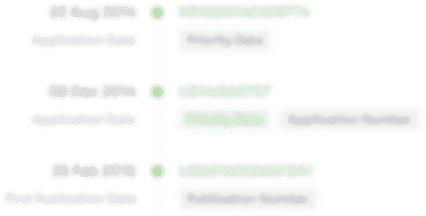
- R&D
- Intellectual Property
- Life Sciences
- Materials
- Tech Scout
- Unparalleled Data Quality
- Higher Quality Content
- 60% Fewer Hallucinations
Browse by: Latest US Patents, China's latest patents, Technical Efficacy Thesaurus, Application Domain, Technology Topic, Popular Technical Reports.
© 2025 PatSnap. All rights reserved.Legal|Privacy policy|Modern Slavery Act Transparency Statement|Sitemap|About US| Contact US: help@patsnap.com