Binocular stereo matching method based on joint up-sampling convolutional neural network
A binocular stereo matching and convolutional neural network technology, applied in the field of computer vision, can solve problems such as easy loss of fine image structure information, inaccurate prediction of target boundaries or parallax results of small-sized objects, etc. The effect of reducing precision and improving computational efficiency
- Summary
- Abstract
- Description
- Claims
- Application Information
AI Technical Summary
Problems solved by technology
Method used
Image
Examples
Embodiment Construction
[0032] The present invention will be further described in detail below in conjunction with the accompanying drawings and embodiments.
[0033] Such as Figure 1-6 As shown, after conventional data preprocessing operations such as scrambling, cropping, and normalization are performed on the original input image, the present invention provides a binocular stereo matching method based on a joint upsampling convolutional neural network, which includes features Three steps of extraction, matching cost aggregation and disparity calculation:
[0034] 1) figure 1 It is a schematic diagram of the overall framework of the present invention. The input of the neural network model to complete the binocular stereo matching task is to match the image pair I 1 and I 2 , the output is the target image I 1 The dense disparity information of , that is, the disparity map D. The network will learn a function (model) f satisfying the following relation:
[0035] f(I 1 , I 2 ) = D
[0036]...
PUM
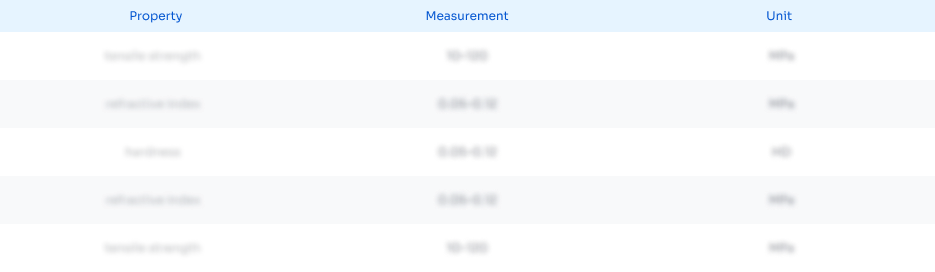
Abstract
Description
Claims
Application Information
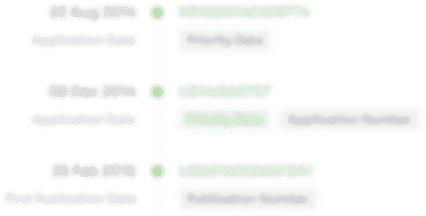
- R&D Engineer
- R&D Manager
- IP Professional
- Industry Leading Data Capabilities
- Powerful AI technology
- Patent DNA Extraction
Browse by: Latest US Patents, China's latest patents, Technical Efficacy Thesaurus, Application Domain, Technology Topic, Popular Technical Reports.
© 2024 PatSnap. All rights reserved.Legal|Privacy policy|Modern Slavery Act Transparency Statement|Sitemap|About US| Contact US: help@patsnap.com