A Brain Tumor Segmentation Method Based on Multi-Hierarchical Relational Learning Network
A learning network and multi-level technology, applied in neural learning methods, biological neural network models, image analysis, etc., can solve the problem that the segmentation model is easy to fall into local optimum, and achieve the effect of reducing differences and improving effectiveness
- Summary
- Abstract
- Description
- Claims
- Application Information
AI Technical Summary
Problems solved by technology
Method used
Image
Examples
Embodiment Construction
[0047] In order to describe the present invention more specifically, the technical solutions of the present invention will be described in detail below with reference to the accompanying drawings and specific embodiments.
[0048] figure 1 It is the low image contrast and fuzzy mode brain tumor segmentation provided by the embodiment of the present invention. The 4th column on the left is the input image of the MRI data, and the 5th column is the corresponding real calibration result. Green, yellow and red areas highlight the WholeTumor (WT), Tumor Core (TC), Enhancing Tumor (ET) sections, respectively.
[0049] figure 2 is the method framework of the present invention. The results are inferred from the modalities using a cascade structure. E and D represent the encoder and decoder in 3D U-Net, respectively, and C is the environment mining module of the method.
[0050] like figure 2 What is shown is that in this paper, a multi-level structure network for segmenting b...
PUM
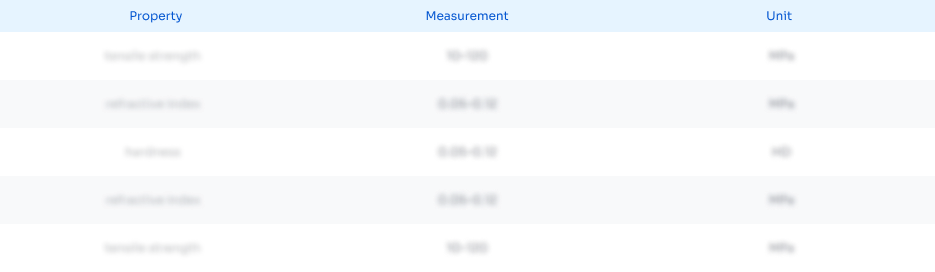
Abstract
Description
Claims
Application Information
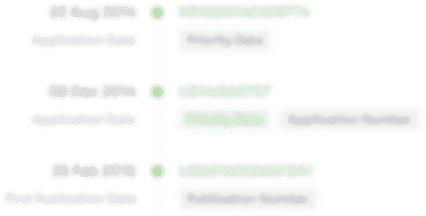
- R&D Engineer
- R&D Manager
- IP Professional
- Industry Leading Data Capabilities
- Powerful AI technology
- Patent DNA Extraction
Browse by: Latest US Patents, China's latest patents, Technical Efficacy Thesaurus, Application Domain, Technology Topic, Popular Technical Reports.
© 2024 PatSnap. All rights reserved.Legal|Privacy policy|Modern Slavery Act Transparency Statement|Sitemap|About US| Contact US: help@patsnap.com