Zero-sample cross-modal retrieval method based on multi-modal feature synthesis
A multi-modal, cross-modal technology, applied in the field of cross-modal retrieval, can solve the problems of ignoring mutual correlation and not optimizing the cross-modal retrieval problem.
- Summary
- Abstract
- Description
- Claims
- Application Information
AI Technical Summary
Problems solved by technology
Method used
Image
Examples
Embodiment
[0058] figure 1 It is a flowchart of a zero-sample cross-modal retrieval method based on multimodal feature synthesis in the present invention.
[0059] In this example, if figure 1 As shown, a zero-sample cross-modal retrieval method based on multimodal feature synthesis of the present invention comprises the following steps:
[0060] S1. Extract multimodal data features
[0061] Multimodal data includes images, text, etc. These raw data are expressed in a way that humans can accept, but computers cannot directly process them. Their features need to be extracted and expressed in numbers that computers can process.
[0062] Download N sets of multimodal data containing images, texts, and image and text shared category labels. These data belong to C categories, and images and texts under each category have shared category labels. Then use the convolutional neural network VGG Net to extract image features v i , using network Doc2vec to extract text features t i , using the ...
PUM
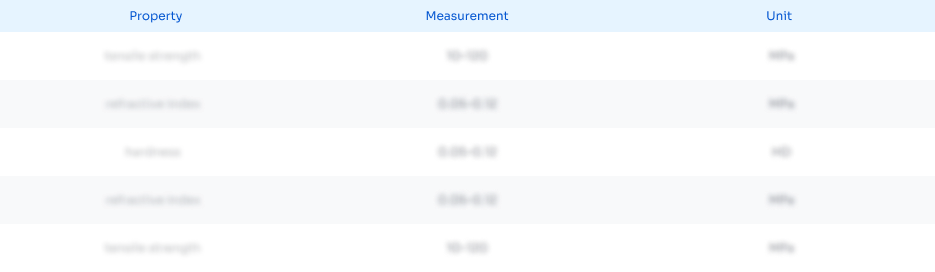
Abstract
Description
Claims
Application Information
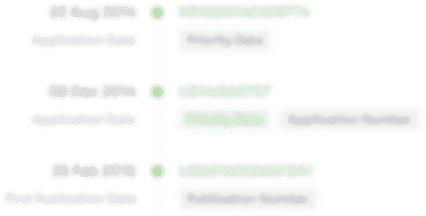
- R&D
- Intellectual Property
- Life Sciences
- Materials
- Tech Scout
- Unparalleled Data Quality
- Higher Quality Content
- 60% Fewer Hallucinations
Browse by: Latest US Patents, China's latest patents, Technical Efficacy Thesaurus, Application Domain, Technology Topic, Popular Technical Reports.
© 2025 PatSnap. All rights reserved.Legal|Privacy policy|Modern Slavery Act Transparency Statement|Sitemap|About US| Contact US: help@patsnap.com