Adaboost ensemble learning power grid fault diagnosis system and method based on data resampling
An integrated learning and power grid fault technology, applied in nuclear methods, data processing applications, instruments, etc., can solve problems such as incomplete fault data, deletion of important sample information, high cost of fault data misclassification, and achieve accurate fault diagnosis and prediction , Accurate fault diagnosis, and the effect of reducing imbalance
- Summary
- Abstract
- Description
- Claims
- Application Information
AI Technical Summary
Problems solved by technology
Method used
Image
Examples
Embodiment Construction
[0025] The technical solutions in the embodiments of the present invention will be clearly and completely described below in conjunction with the accompanying drawings.
[0026] Such as figure 1 with figure 2 As shown, a kind of Adaboost integrated learning grid fault diagnosis system based on data resampling, the system includes a fault information database 1, a data preprocessing module 2 and a fault diagnosis module 3, the fault information database 1, data preprocessing module 2 All are connected with the fault diagnosis module 3 through network equipment, the fault information database 1 is used to store fault information packets; the data preprocessing module 2 is used to fill in vacant values and normalize the data; The fault diagnosis module 3 includes a data resampling module 301, a decision tree base classifier module 302 and an Adaboost integrated classifier module 303, and the data resampling module 301 is used to balance the data; The classifier module 302 is...
PUM
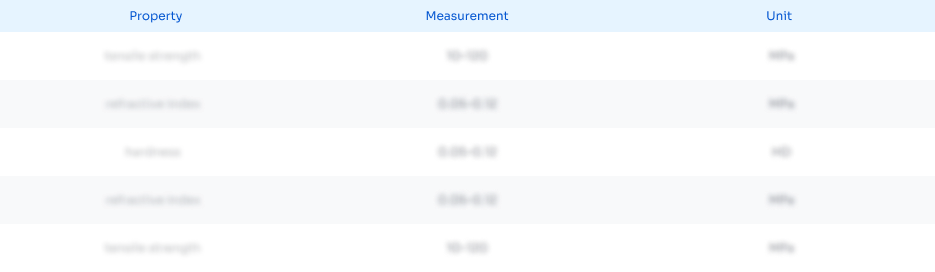
Abstract
Description
Claims
Application Information
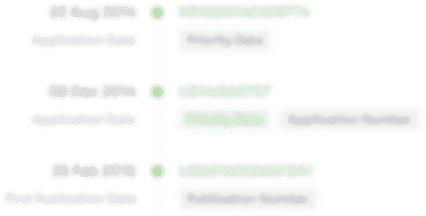
- R&D
- Intellectual Property
- Life Sciences
- Materials
- Tech Scout
- Unparalleled Data Quality
- Higher Quality Content
- 60% Fewer Hallucinations
Browse by: Latest US Patents, China's latest patents, Technical Efficacy Thesaurus, Application Domain, Technology Topic, Popular Technical Reports.
© 2025 PatSnap. All rights reserved.Legal|Privacy policy|Modern Slavery Act Transparency Statement|Sitemap|About US| Contact US: help@patsnap.com