Building electrical load comprehensive prediction method and system based on LSTM neural network
A neural network and comprehensive prediction technology, applied in biological neural network models, predictions, neural architectures, etc., can solve problems such as difficulty in providing prediction accuracy, and achieve the effect of easy implementation and high accuracy
- Summary
- Abstract
- Description
- Claims
- Application Information
AI Technical Summary
Problems solved by technology
Method used
Image
Examples
Embodiment 1
[0035] See attached figure 2 As shown, this embodiment discloses a comprehensive prediction method for building electrical load based on LSTM neural network. Building electrical load planning is the most important in comprehensive energy planning. The electrical load conditions of different geographical locations and different types of buildings are not only It is not the same, but also need to consider various influencing factors such as building area, climate conditions in the area where the building is located, flow of people in the building, work and rest time, and weather factors.
[0036] Power consumption data include: inherent information such as building type, building area, functional zoning, geographical location, and climate conditions, building flow of people, work and rest time, and corresponding meteorological data (including temperature, humidity, etc.), historical load information including electric load curves, electricity consumption, etc. curve. The LSTM ...
Embodiment 2
[0086] Based on the same inventive concept, the purpose of this embodiment is to provide a computing device, which includes a memory, a processor, and a computer program stored in the memory and operable on the processor. The steps of a comprehensive forecasting method for building electrical load based on LSTM neural network in Example 1.
Embodiment 3
[0088] Based on the same inventive concept, the purpose of this embodiment is to provide a computer-readable storage medium.
[0089] A computer-readable storage medium, on which a computer program is stored, and when the program is executed by a processor, the steps of the method for building electrical load comprehensive forecasting based on LSTM neural network in Embodiment 1 are executed.
[0090] Embodiment three
[0091] Based on the same inventive concept, the purpose of this embodiment is to provide a building electrical load comprehensive forecasting system based on LSTM neural network.
[0092] A building electrical load comprehensive forecasting system based on LSTM neural network, including:
[0093] Data processing module: obtain load data, weather parameters and building data of typical buildings and perform normalization processing;
[0094] LSTM forecasting model training module: establish the electrical load forecasting model of the LSTM neural network, dete...
PUM
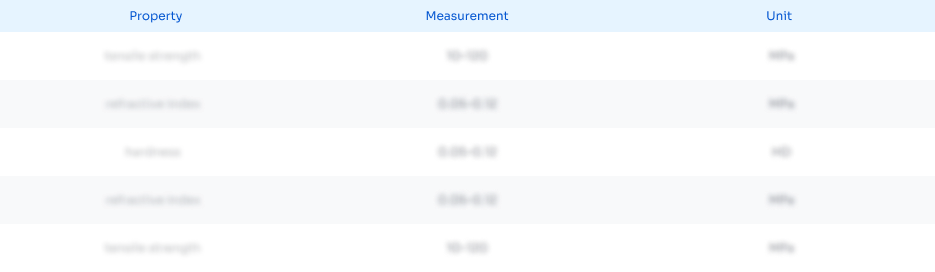
Abstract
Description
Claims
Application Information
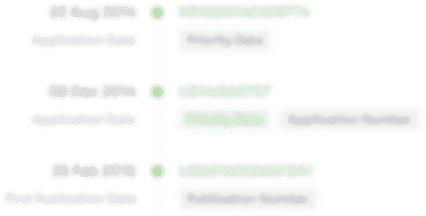
- Generate Ideas
- Intellectual Property
- Life Sciences
- Materials
- Tech Scout
- Unparalleled Data Quality
- Higher Quality Content
- 60% Fewer Hallucinations
Browse by: Latest US Patents, China's latest patents, Technical Efficacy Thesaurus, Application Domain, Technology Topic, Popular Technical Reports.
© 2025 PatSnap. All rights reserved.Legal|Privacy policy|Modern Slavery Act Transparency Statement|Sitemap|About US| Contact US: help@patsnap.com