Cross-modal retrieval method based on graph convolutional neural network
A convolutional neural network and cross-modal technology, applied in the field of end-to-end cross-modal retrieval, can solve the problems of insufficient utilization of multi-modal data, poor data representation ability, and low retrieval accuracy, and achieve cross-modal The effect of strong retrieval and representation ability and narrowing the semantic gap
- Summary
- Abstract
- Description
- Claims
- Application Information
AI Technical Summary
Problems solved by technology
Method used
Image
Examples
Embodiment Construction
[0032] In order to make the objectives, technical solutions and advantages of the present invention clearer, the present invention will be further described below in conjunction with specific embodiments and with reference to the accompanying drawings.
[0033] Reference figure 1 , A cross-modal retrieval method based on graph convolutional neural networks, including four processes: network construction, data set preprocessing, network training, retrieval and accuracy testing.
[0034] The multi-modal data set used in this implementation case contains a total of 4,500 pairs of multi-modal data, and each pair of multi-modal data includes an image, a set of image-related data, a paragraph of text, and a set of text-related data. Each pair is marked with a category label, and the label has three categories.
[0035] The cross-modal retrieval method based on graph convolutional neural network includes the following steps:
[0036] Step 1. Network construction, the process is as follows: ...
PUM
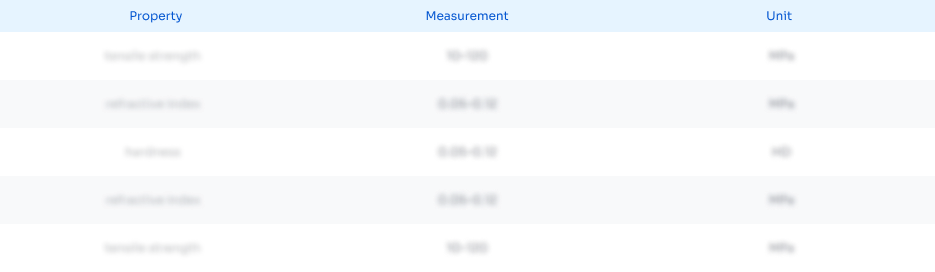
Abstract
Description
Claims
Application Information
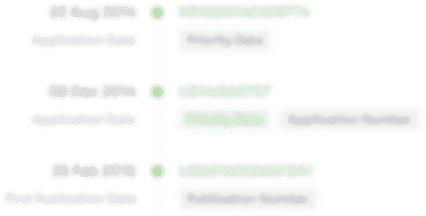
- R&D
- Intellectual Property
- Life Sciences
- Materials
- Tech Scout
- Unparalleled Data Quality
- Higher Quality Content
- 60% Fewer Hallucinations
Browse by: Latest US Patents, China's latest patents, Technical Efficacy Thesaurus, Application Domain, Technology Topic, Popular Technical Reports.
© 2025 PatSnap. All rights reserved.Legal|Privacy policy|Modern Slavery Act Transparency Statement|Sitemap|About US| Contact US: help@patsnap.com