Decentralized federated machine learning method under privacy protection
A decentralized and privacy-protecting technology, applied in the intersecting field of machine learning and information security, can solve problems such as user attack behavior, attacks, and participant data leakage that are not actually taken into account
- Summary
- Abstract
- Description
- Claims
- Application Information
AI Technical Summary
Problems solved by technology
Method used
Image
Examples
Embodiment Construction
[0081] In order to make the object, technical solution and advantages of the present invention clearer, the present invention will be further described in detail below in conjunction with the accompanying drawings and embodiments. It should be understood that the specific embodiments described here are only used to explain the present invention, not to limit the present invention. In addition, the technical features involved in the various embodiments of the present invention described below can be combined with each other as long as they do not constitute a conflict with each other.
[0082] The present invention takes image recognition in the field of machine learning as an example, and deploys image recognition tasks in the decentralized federated learning under privacy protection of the present invention. In order to compare the difference between the present invention and the general machine learning implementation, the present invention will use centralized machine learn...
PUM
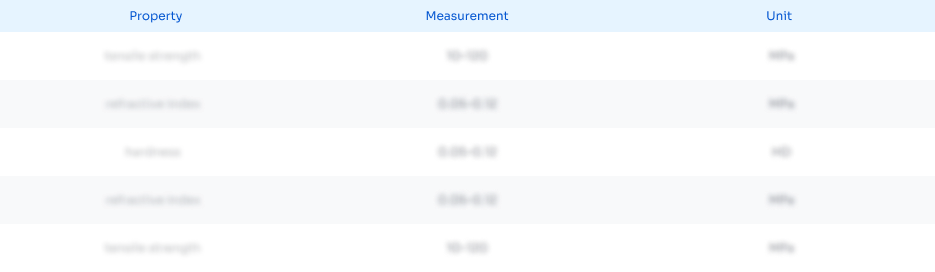
Abstract
Description
Claims
Application Information
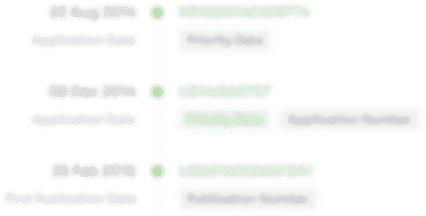
- R&D Engineer
- R&D Manager
- IP Professional
- Industry Leading Data Capabilities
- Powerful AI technology
- Patent DNA Extraction
Browse by: Latest US Patents, China's latest patents, Technical Efficacy Thesaurus, Application Domain, Technology Topic.
© 2024 PatSnap. All rights reserved.Legal|Privacy policy|Modern Slavery Act Transparency Statement|Sitemap