Tumble behavior analysis and detection method based on double-flow convolutional neural network
A convolutional neural network and behavior analysis technology, applied in the field of fall behavior analysis and detection, can solve the problems of unachievable speed and accuracy, unsatisfactory neural network effect, etc., achieve less calculation, reduce hardware equipment cost, and broad The effect of market prospects
- Summary
- Abstract
- Description
- Claims
- Application Information
AI Technical Summary
Problems solved by technology
Method used
Image
Examples
Embodiment Construction
[0035] The present invention will be described in further detail below in conjunction with the accompanying drawings. The present invention provides a fall behavior analysis and detection method based on a two-stream convolutional neural network, such as figure 1 As shown in , it is a schematic diagram of the analysis and detection model architecture, which specifically includes the following steps:
[0036] Step 1: Extract pedestrian profile images and motion history maps to form a falls dataset.
[0037] Motion History Image (MHI) is a grayscale image that can represent motion information. By calculating the change of pixel grayscale value at the same position within a time period, the target motion is expressed in the form of image grayscale value. The higher the gray value of the pixel, the closer the motion occurred. Therefore, the motion history map contains the target motion direction information. Since the gradient direction corresponds to the direction in which the...
PUM
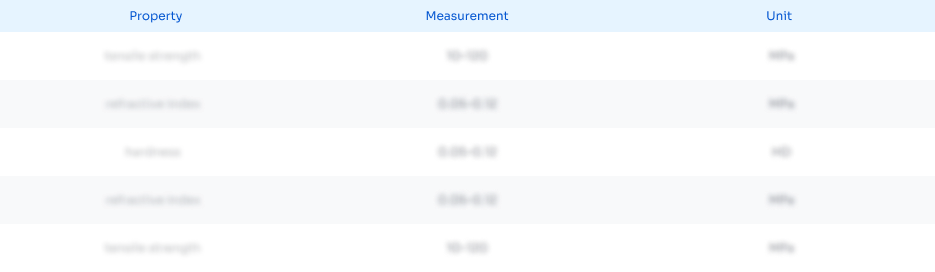
Abstract
Description
Claims
Application Information
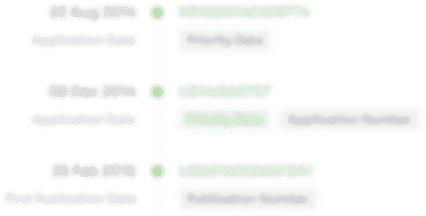
- Generate Ideas
- Intellectual Property
- Life Sciences
- Materials
- Tech Scout
- Unparalleled Data Quality
- Higher Quality Content
- 60% Fewer Hallucinations
Browse by: Latest US Patents, China's latest patents, Technical Efficacy Thesaurus, Application Domain, Technology Topic, Popular Technical Reports.
© 2025 PatSnap. All rights reserved.Legal|Privacy policy|Modern Slavery Act Transparency Statement|Sitemap|About US| Contact US: help@patsnap.com