Water environment remote sensing data modeling method based on multilayer convolutional neural network
A convolutional neural network and remote sensing data technology, which is applied in the field of water environment remote sensing data modeling based on multi-layer convolutional neural networks, can solve the problems of time-consuming, inability to perform real-time monitoring, and inability to fully reflect the overall temporal and spatial changes of the water ecological environment. , to reduce the complexity
- Summary
- Abstract
- Description
- Claims
- Application Information
AI Technical Summary
Problems solved by technology
Method used
Image
Examples
Embodiment 1
[0032] Such as Figure 1-4 As shown, the HJ-1A / B satellite was successfully launched at 11:25 am on September 6, 2008. The design principles of the two CCD cameras mounted on the two satellites are exactly the same, and can generate push-broom imaging in four spectral bands , the ground swath width is 700 kilometers, the ground pixel resolution is 30 meters, and the revisit period after the two CCD cameras are networked is only 2 days, and the transit time of Changshou Lake is about 10-11a.m. From March 2015 to October 2018, 157 remote sensing images were actually acquired. During the field sampling period, the HJ-1 / CCD remote sensing images, the lake area is 66km2, and one image can cover it.
[0033] Before using the multi-layer convolutional neural network algorithm to train the image, the image is preprocessed such as normalization, and the data is mapped to the activation function value range (0,1) of the output layer. Due to the difficulty in obtaining remote sensing im...
Embodiment 2
[0036] Such as Figure 5 As shown in the figure, it shows the change of water eutrophication in a remote sensing image of Changshou Lake in different periods in 2018. It can be seen from the figure that the data presented after the convolution layer of the remote sensing image can be displayed more clearly and intuitively . In the figure, the chlorophyll concentration of the water body began to increase in April and reached its peak in July. This is consistent with the measured results. Therefore, after repeated trials and comparisons, the convolutional neural network algorithm for water environment remote sensing based on multi-layer convolutional neural networks can extract remote sensing information more accurately, and can basically meet the requirements of automatic inversion of water quality status.
PUM
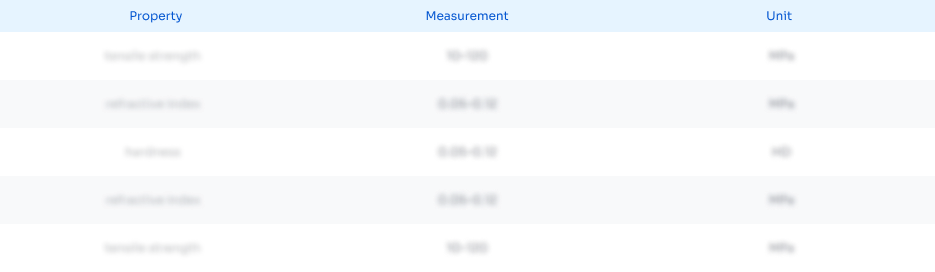
Abstract
Description
Claims
Application Information
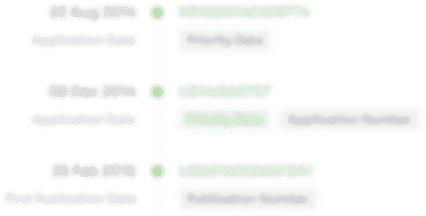
- R&D Engineer
- R&D Manager
- IP Professional
- Industry Leading Data Capabilities
- Powerful AI technology
- Patent DNA Extraction
Browse by: Latest US Patents, China's latest patents, Technical Efficacy Thesaurus, Application Domain, Technology Topic, Popular Technical Reports.
© 2024 PatSnap. All rights reserved.Legal|Privacy policy|Modern Slavery Act Transparency Statement|Sitemap|About US| Contact US: help@patsnap.com