Mechanical fault diagnosis method based on migration relation network
A relational network and mechanical fault technology, applied in the field of machine learning, can solve problems such as difficulty in adapting and meeting the application requirements of intelligent diagnosis of mechanical faults, low accuracy of deep migration diagnosis, and ignoring the degree of correlation between source machinery and target machinery of diagnosis knowledge.
- Summary
- Abstract
- Description
- Claims
- Application Information
AI Technical Summary
Problems solved by technology
Method used
Image
Examples
Embodiment Construction
[0060] The specific implementation manners of the present invention will be further described in detail below in conjunction with the accompanying drawings and examples. The following implementations are used to explain the present invention, but not to limit the scope of the present invention.
[0061] like figure 1 Shown, the present invention is based on the mechanical diagnosis method of migration relationship network, comprises the following steps:
[0062] Step 1: Transfer learning is a machine learning method that uses learned knowledge to solve problems in different but related fields. Simply put, it is the knowledge learned in a field, and the application of a new neighborhood is completed through the method of transfer learning. Therefore, different from traditional deep learning, deep transfer learning samples require source domain data and target domain data. Using existing source domain data to solve the target domain problem with scarce samples in engineering p...
PUM
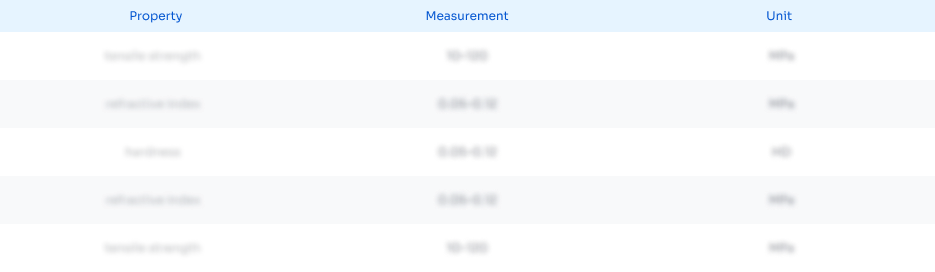
Abstract
Description
Claims
Application Information
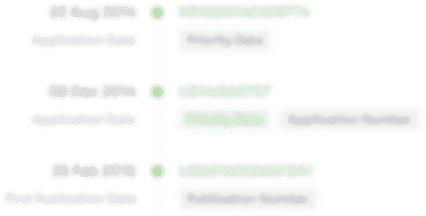
- R&D Engineer
- R&D Manager
- IP Professional
- Industry Leading Data Capabilities
- Powerful AI technology
- Patent DNA Extraction
Browse by: Latest US Patents, China's latest patents, Technical Efficacy Thesaurus, Application Domain, Technology Topic, Popular Technical Reports.
© 2024 PatSnap. All rights reserved.Legal|Privacy policy|Modern Slavery Act Transparency Statement|Sitemap|About US| Contact US: help@patsnap.com