Representation learning method based on superimposed convolution sparse auto-encoder
A sparse autoencoder and learning method technology, applied in the field of representation learning based on superimposed convolutional sparse autoencoders, can solve the problems of high-dimensional feature representations that are not abstract and robust enough, errors, and model performance degradation
- Summary
- Abstract
- Description
- Claims
- Application Information
AI Technical Summary
Problems solved by technology
Method used
Image
Examples
Embodiment Construction
[0067] Such as figure 1 A representation learning method based on stacked convolutional sparse autoencoders is shown, including:
[0068] Step 1) Design and implement a reconstruction independent component analysis algorithm including whitening, and use the image data set as input, iteratively optimize and learn the output reconstruction matrix, and obtain the trained sparse autoencoder model;
[0069] Step 2) building a semi-supervised superposition sparse autoencoder model to train the feature representation;
[0070] Step 3) Build a convolutional model to extract block features from the data, apply convolution and pooling operations to generate convolutional feature representations;
[0071] Step 4) superimposing the convolutional sparse autoencoder to further optimize the convolutional feature representation;
[0072] Step 5) On the basis of the finally learned feature representation, use the logistic regression model to train a classifier on the image data set, and obta...
PUM
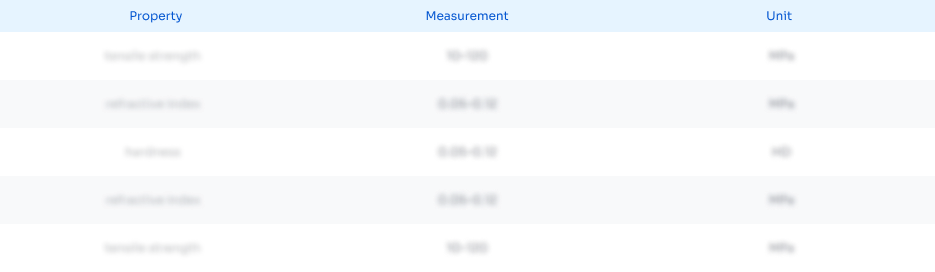
Abstract
Description
Claims
Application Information
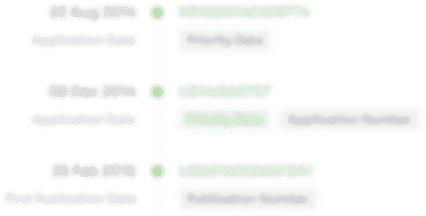
- R&D
- Intellectual Property
- Life Sciences
- Materials
- Tech Scout
- Unparalleled Data Quality
- Higher Quality Content
- 60% Fewer Hallucinations
Browse by: Latest US Patents, China's latest patents, Technical Efficacy Thesaurus, Application Domain, Technology Topic, Popular Technical Reports.
© 2025 PatSnap. All rights reserved.Legal|Privacy policy|Modern Slavery Act Transparency Statement|Sitemap|About US| Contact US: help@patsnap.com