Self-acceptance technology debt detection and classification method based on multi-method ensemble learning
A technology integrating learning and classification methods, applied in the field of self-admitted debt classification and detection, can solve the problems of low classifier performance, poor flexibility, low efficiency, etc., to achieve the effect of optimizing prediction results, improving detection indicators, and improving the performance of training classifiers
- Summary
- Abstract
- Description
- Claims
- Application Information
AI Technical Summary
Problems solved by technology
Method used
Image
Examples
Embodiment Construction
[0050] Now in conjunction with embodiment, accompanying drawing, the present invention will be further described:
[0051] The invention is a self-recognition technology detection and classification method based on multi-method integrated learning. The method mainly includes five core steps: preprocessing the feature words; selecting the top k most useful features to train the classifier; using the naive Bayesian polynomial ( Bayes Multinomial) and Linear Logistic Regression (Simple Logistic) two methods to train the corresponding sub-classifiers; and integrate the prediction results through the sub-classifier voting rules to obtain precision, recall, and comprehensive accuracy And the recall rate finally calculates the F1 value (F1-score) as a subsequent evaluation standard. Finally, the clustering method is used to cluster the features that often appear in the experiment process and have high information gain value, and then classify the detected technical debt.
[0052] ...
PUM
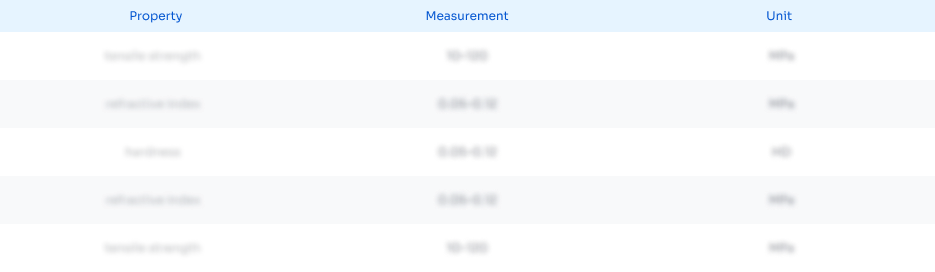
Abstract
Description
Claims
Application Information
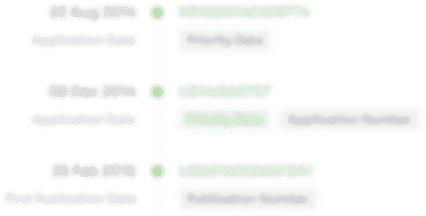
- R&D Engineer
- R&D Manager
- IP Professional
- Industry Leading Data Capabilities
- Powerful AI technology
- Patent DNA Extraction
Browse by: Latest US Patents, China's latest patents, Technical Efficacy Thesaurus, Application Domain, Technology Topic, Popular Technical Reports.
© 2024 PatSnap. All rights reserved.Legal|Privacy policy|Modern Slavery Act Transparency Statement|Sitemap|About US| Contact US: help@patsnap.com