Hierarchical compressed image matching method and system based on orthogonal attention mechanism
A matching method and attention technology, applied in the field of graph computing and knowledge graph, can solve problems such as high time complexity and unbearable time
- Summary
- Abstract
- Description
- Claims
- Application Information
AI Technical Summary
Problems solved by technology
Method used
Image
Examples
Embodiment 1
[0081] Figure 1 to Figure 5 Shows a specific embodiment of the present invention, a hierarchical compression image matching method based on an orthogonal attention mechanism, which is mainly aimed at the matching problem of large images, such as figure 1 As shown, the logistic regression model used in this embodiment according to the scale of the processed data graph includes the following steps:
[0082] Step 1: Obtain the big picture data pair to be matched, and preprocess the big picture data. The preprocessing refers to the point vector initialization of the graph. The big picture data refers to the graph with more than 16 points; In this embodiment, a large picture from 20 to 500 points is used;
[0083] Step 2: Train the large-picture matching model based on the orthogonal attention mechanism according to the historical gallery; the specific training method of the large-picture matching model is:
[0084] Step 2.1: Obtain all the big picture data in the historical gallery, an...
Embodiment 2
[0117] The difference from the first embodiment is that this embodiment is mainly for the matching problem of small graphs, that is, for graphs with nodes less than 16 points, for small graphs, the prior art uses A* algorithm for graph matching However, this embodiment uses two layers of orthogonal attention and graph attention compression, and uses the compressed graph for graph matching.
[0118] A hierarchical compression graph matching method based on orthogonal attention mechanism, such as Image 6 As shown, including the following steps:
[0119] S1: Obtain the three-tuple graph data to be matched, and perform preprocessing on the small graph data. The preprocessing refers to the point vector initialization of the graph, and the small graph data refers to a graph whose number of nodes is less than 16 points;
[0120] S2: Train a small image matching model based on the orthogonal attention mechanism according to the historical gallery;
[0121] According to the method of claim 5,...
PUM
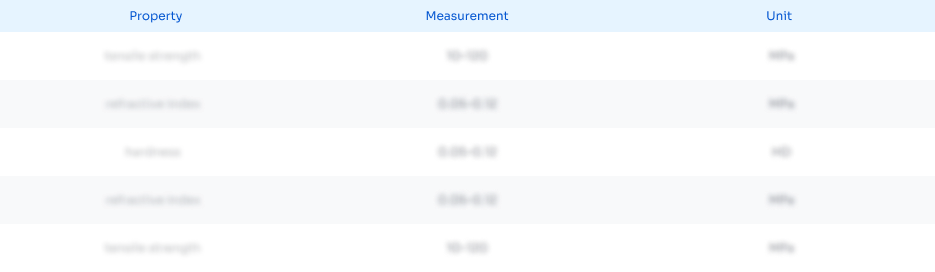
Abstract
Description
Claims
Application Information
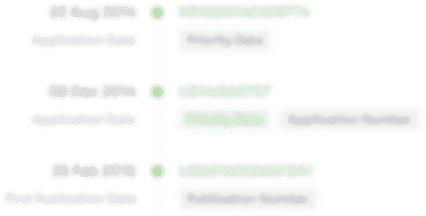
- R&D Engineer
- R&D Manager
- IP Professional
- Industry Leading Data Capabilities
- Powerful AI technology
- Patent DNA Extraction
Browse by: Latest US Patents, China's latest patents, Technical Efficacy Thesaurus, Application Domain, Technology Topic, Popular Technical Reports.
© 2024 PatSnap. All rights reserved.Legal|Privacy policy|Modern Slavery Act Transparency Statement|Sitemap|About US| Contact US: help@patsnap.com