MRI segmentation method based on reinforcement learning multi-scale neural network
A multi-scale segmentation and reinforcement learning technology, applied in the field of image processing, can solve the problems of inconsistent judgment severity, difference in MRI data quality, and inability to quantify, and achieve the effect of improving segmentation effect, improving segmentation accuracy, and enhancing learning.
- Summary
- Abstract
- Description
- Claims
- Application Information
AI Technical Summary
Problems solved by technology
Method used
Image
Examples
Embodiment 1
[0025] With the development of science and technology, human beings have a better understanding of spinal arthritis. More information about the inflammatory areas of spinal arthritis can be found through single-mode MRI images. In the region segmentation, the segmentation effect is often poor due to the large difference in the shape and size of the inflammatory region in the MRI image data. Aiming at the current situation, the present invention proposes an MRI segmentation method based on reinforcement learning multi-scale neural network after exploration and experimentation, which is used for segmentation of inflammatory regions in single-modality MRI images.
[0026] The invention is an MRI segmentation method based on multi-scale neural network of reinforcement learning.
[0027] see figure 1 , including the following steps:
[0028] (1) Divide the training, verification and testing sample sets: Firstly, the original MRI data of AS patients were obtained from the hospital...
Embodiment 2
[0039] The MRI segmentation method based on reinforcement learning multi-scale neural network is the same as that in Embodiment 1, and the voxel constraint strategy described in step (2) of the present invention sets the label value of the MRI inflammation region. The voxel constraint strategy proposed by the present invention is aimed at the problem of poor segmentation effect caused by large differences in the distribution of voxel values in the inflammatory region. The label value of the inflammatory region is modified according to the voxel value of the MRI data, and the label value of the inflammatory region is adaptively adjusted. According to the voxel value of the inflammatory area, it is adjusted as follows:
[0040]
[0041]
[0042] Modify the label value of the original inflammatory area according to the voxel value of the MRI data through the above formula, where y n is the original label value, y′ n is the modified label value, σ is the weighted value, p...
Embodiment 3
[0046] The MRI segmentation method based on reinforcement learning multi-scale neural network is the same as embodiment 1-2, and the construction described in the step (3) of the present invention is used to deal with the MRI based on reinforcement learning multi-scale neural network for processing large differences in shape and size and diffuse fuzzy inflammatory regions The segmentation model LGR-Net, which addresses the segmentation of multi-scale and diffusely ambiguous inflammatory regions. Including the following steps:
[0047] (3.1) Constructing a multi-scale segmentation sub-network: Firstly, aiming at the problem of large differences in the shape and size of inflammatory regions, a multi-scale convolution module GMS is constructed to extract multi-scale information. Considering the network size limitation and the commonly used convolution kernel size, the design Nine commonly used convolution kernels with different hole rates d and different sizes k are connected in ...
PUM
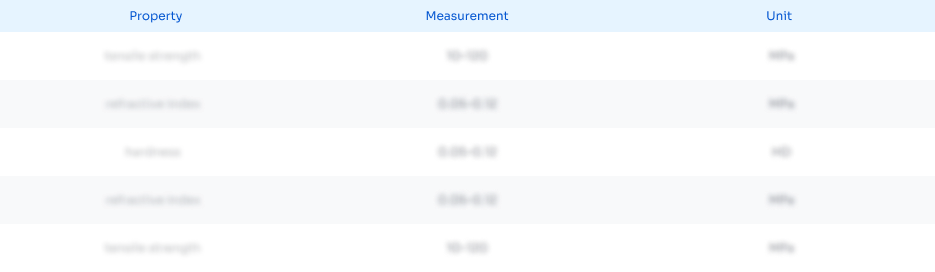
Abstract
Description
Claims
Application Information
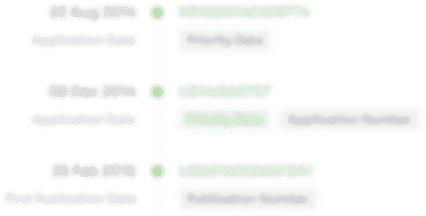
- Generate Ideas
- Intellectual Property
- Life Sciences
- Materials
- Tech Scout
- Unparalleled Data Quality
- Higher Quality Content
- 60% Fewer Hallucinations
Browse by: Latest US Patents, China's latest patents, Technical Efficacy Thesaurus, Application Domain, Technology Topic, Popular Technical Reports.
© 2025 PatSnap. All rights reserved.Legal|Privacy policy|Modern Slavery Act Transparency Statement|Sitemap|About US| Contact US: help@patsnap.com