Fixed-point quantitative convolutional neural network accelerator calculation circuit
A convolutional neural network, computing circuit technology, applied in the field of integrated circuits
- Summary
- Abstract
- Description
- Claims
- Application Information
AI Technical Summary
Problems solved by technology
Method used
Image
Examples
Embodiment Construction
[0034] The technical solution of the present invention will be described in detail below in conjunction with the accompanying drawings and specific embodiments.
[0035] The present invention proposes a convolutional neural network accelerator computing circuit based on fixed-point quantization. The application of convolutional neural network with INT8 integer quantization and the quantization specification of TensorFlow for quantization scheme selection are used as examples to illustrate, but the specific quantization types and quantization schemes should not be construed as a limitation of the invention. First, quantize the INT8 data type of the convolutional neural network. On this basis, the accelerator computing circuit proposed by the present invention is applied. After shaping and quantizing, not only the memory space occupied by the weight becomes 1 / 4 of the original, but also more can be transmitted under the same bandwidth. Multi-data, and the calculation process is ...
PUM
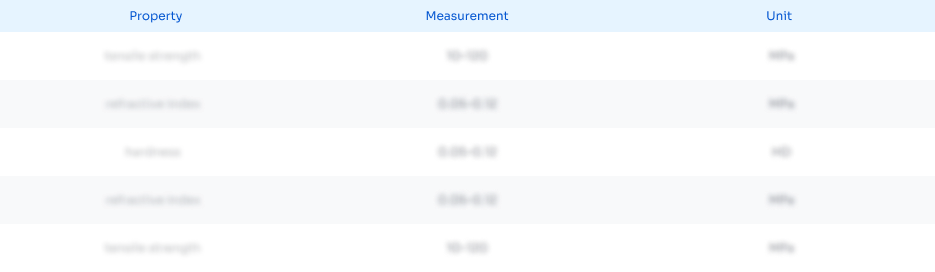
Abstract
Description
Claims
Application Information
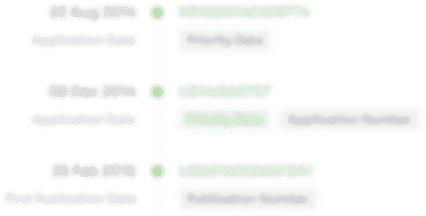
- R&D
- Intellectual Property
- Life Sciences
- Materials
- Tech Scout
- Unparalleled Data Quality
- Higher Quality Content
- 60% Fewer Hallucinations
Browse by: Latest US Patents, China's latest patents, Technical Efficacy Thesaurus, Application Domain, Technology Topic, Popular Technical Reports.
© 2025 PatSnap. All rights reserved.Legal|Privacy policy|Modern Slavery Act Transparency Statement|Sitemap|About US| Contact US: help@patsnap.com