Mobile terminal disease intelligent auxiliary diagnosis system based on deep learning
A deep learning and auxiliary diagnosis technology, applied in the field of mobile medicine, can solve the problems of low repeatability, high subjectivity of manual reading, time-consuming and labor-intensive knowledge and experience inheritance difficulties, etc., to improve efficiency and accuracy, and improve disease diagnosis. Precise results
- Summary
- Abstract
- Description
- Claims
- Application Information
AI Technical Summary
Problems solved by technology
Method used
Image
Examples
Embodiment 1
[0069] figure 1 , 2As shown, the system is used to distinguish between benign and malignant pulmonary nodules in CT images. First, collect DICOM images of the lungs with pathological results, analyze the DICOM images, and obtain the grayscale information of the file and the included image pixel information with a single template. The gray value of the image is normalized. At the same time, in order to prevent the phenomenon of network overfitting, it is necessary to increase the number of training images, randomly rotate, flip, distort, adjust the contrast, brightness and other enhancements and disturbance data preprocessing operations on the image; secondly , extract the two-dimensional features of pulmonary nodules under the multi-scale view from the underlying pixel-level information. According to the size of the image, the size of the convolution kernel and the depth of the network are reasonably selected. In order to enhance the performance of the model and reduce the num...
Embodiment 2
[0072] figure 1 , 2 As shown, the system is used for pathological analysis of CT images of lung tumors. First, collect DICOM images of lungs with pathological results, analyze the DICOM images of lungs, obtain file grayscale information with multiple templates, and select the lesion area to determine Image serial number, in order to reduce the phenomenon of network over-fitting, it is necessary to increase the number of training images, perform image rotation, mirroring, increase white noise and other enhancements and disturb data preprocessing operations; secondly, in order to solve the problem of training sample images due to human intervention. Few and deep learning models require a large number of contradictions between samples. A transfer learning (Transfer Learning) network model is proposed to extract features from training samples, and then encode them into low-dimensional feature vectors while retaining feature information as much as possible and greatly reduce the de...
PUM
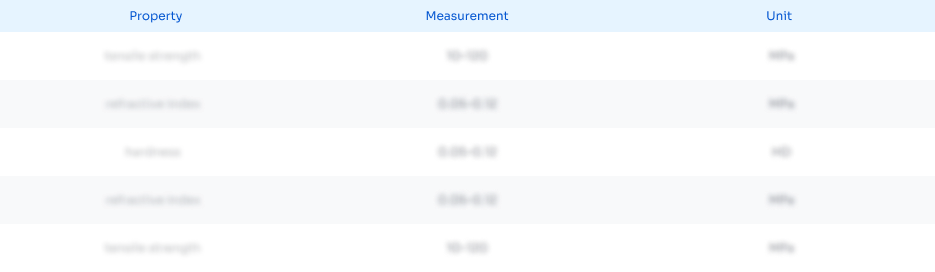
Abstract
Description
Claims
Application Information
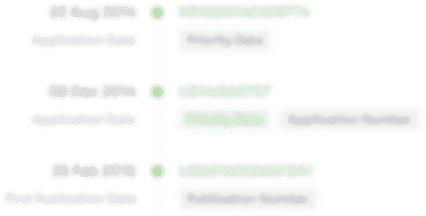
- R&D
- Intellectual Property
- Life Sciences
- Materials
- Tech Scout
- Unparalleled Data Quality
- Higher Quality Content
- 60% Fewer Hallucinations
Browse by: Latest US Patents, China's latest patents, Technical Efficacy Thesaurus, Application Domain, Technology Topic, Popular Technical Reports.
© 2025 PatSnap. All rights reserved.Legal|Privacy policy|Modern Slavery Act Transparency Statement|Sitemap|About US| Contact US: help@patsnap.com