Complex manufacturing system regression scheduling method
A manufacturing system and scheduling method technology, applied in general control systems, control/regulation systems, program control, etc., can solve problems such as low computational efficiency, excessive computational burden, and difficulty in meeting the multi-objective and multi-constraints of the manufacturing system, and achieve optimization. Network structure, the effect of improving computing efficiency
- Summary
- Abstract
- Description
- Claims
- Application Information
AI Technical Summary
Problems solved by technology
Method used
Image
Examples
Embodiment Construction
[0024] The present invention will be described in detail below with reference to the accompanying drawings and examples.
[0025] Generally speaking, the data-driven dynamic scheduling of complex manufacturing systems can usually be divided into two stages, namely, the offline learning stage of the scheduling model and the online application stage of the scheduling model. Offline learning of the scheduling model, that is, using machine learning methods to analyze historical data according to the scheduling target in an offline state, establishing a scheduling model, and realizing the mapping between production status and scheduling strategy; online application of the scheduling model, that is, applying scheduling during online dynamic scheduling The model gives a scheduling policy that matches the real-time state. A dynamic scheduling framework for complex manufacturing systems such as figure 1 As shown, it consists of four parts: data acquisition, data processing, machine le...
PUM
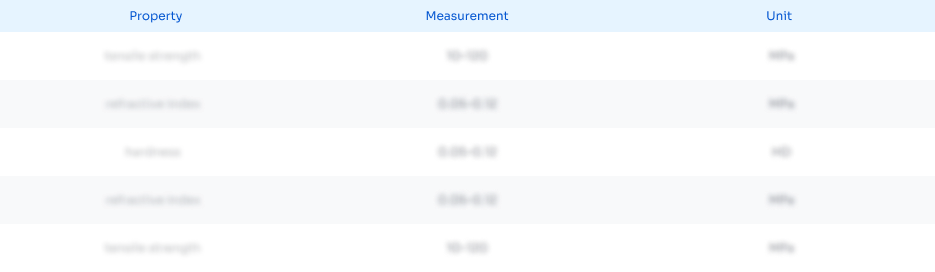
Abstract
Description
Claims
Application Information
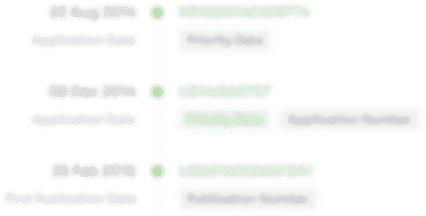
- R&D
- Intellectual Property
- Life Sciences
- Materials
- Tech Scout
- Unparalleled Data Quality
- Higher Quality Content
- 60% Fewer Hallucinations
Browse by: Latest US Patents, China's latest patents, Technical Efficacy Thesaurus, Application Domain, Technology Topic, Popular Technical Reports.
© 2025 PatSnap. All rights reserved.Legal|Privacy policy|Modern Slavery Act Transparency Statement|Sitemap|About US| Contact US: help@patsnap.com