CNN-based width learning classification method
A classification method and width technology, applied in the field of CNN-based breadth learning classification, can solve the problems of classification task accuracy loss, learning ability decline, insufficient sample representative feature description, etc., to achieve good classification results, strong representation ability, high The effect of efficiency
- Summary
- Abstract
- Description
- Claims
- Application Information
AI Technical Summary
Problems solved by technology
Method used
Image
Examples
Embodiment Construction
[0034] The preferred embodiments of the present invention will be described below in conjunction with the accompanying drawings. It should be understood that the preferred embodiments described here are only used to illustrate and explain the present invention, and are not intended to limit the present invention.
[0035] Such as figure 1 As described, it is a CNN-based width learning classification method in a preferred embodiment of the present invention, and the technical solution including the following specific steps is realized:
[0036] Step 1. Obtain training data and test data;
[0037] Step 2. Preprocessing the training data and test data;
[0038] Step 3, using the convolutional neural network (CNN) to extract the features of the training data, obtain the feature map of the training data, and generate the feature node layer of the width learning basic model;
[0039] Step 3-1: For the preprocessed input data X, use the convolutional neural network model to generat...
PUM
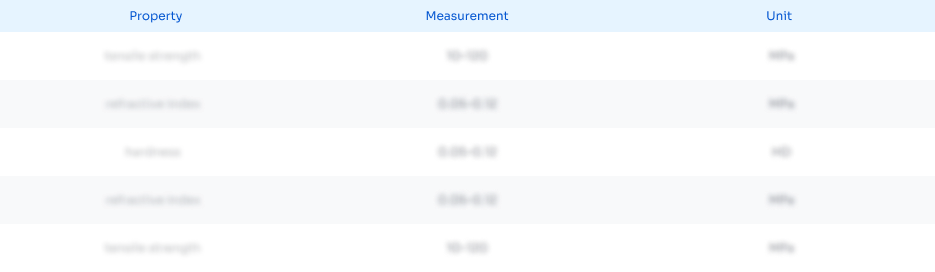
Abstract
Description
Claims
Application Information
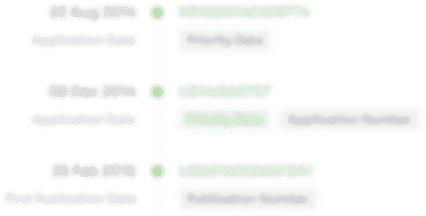
- R&D
- Intellectual Property
- Life Sciences
- Materials
- Tech Scout
- Unparalleled Data Quality
- Higher Quality Content
- 60% Fewer Hallucinations
Browse by: Latest US Patents, China's latest patents, Technical Efficacy Thesaurus, Application Domain, Technology Topic, Popular Technical Reports.
© 2025 PatSnap. All rights reserved.Legal|Privacy policy|Modern Slavery Act Transparency Statement|Sitemap|About US| Contact US: help@patsnap.com