Zero-sample image hash retrieval method based on visual-to-semantic network
A semantic network and sample image technology, applied in still image data retrieval, still image data indexing, neural learning methods, etc., can solve the problems of poor hash algorithm effect, high and low similarity, poor generalization, etc., to reduce training overhead , the effect of good generalization and robustness
- Summary
- Abstract
- Description
- Claims
- Application Information
AI Technical Summary
Problems solved by technology
Method used
Image
Examples
Embodiment Construction
[0040] The preferred embodiments of the present invention will be described below in conjunction with the accompanying drawings. It should be understood that the preferred embodiments described here are only used to illustrate and explain the present invention, and are not intended to limit the present invention.
[0041] In order to achieve the purpose of the present invention, the CUB data set is used for training and retrieval. When cutting the data set, 4000 pictures in 150 categories are used as the training data set 400 images in 50 categories as test data set X Q , and all other pictures are used as the image set X to be retrieved in the database P , and at the same time input the semantic features of 1000 bird label categories The goal of the embodiment is to use a hash algorithm with a specified number of bits, for X Q and X Q is hashed, and from X P Find the most relevant image sorting in , so as to obtain higher MAP and Precision@R indicators. In one of the e...
PUM
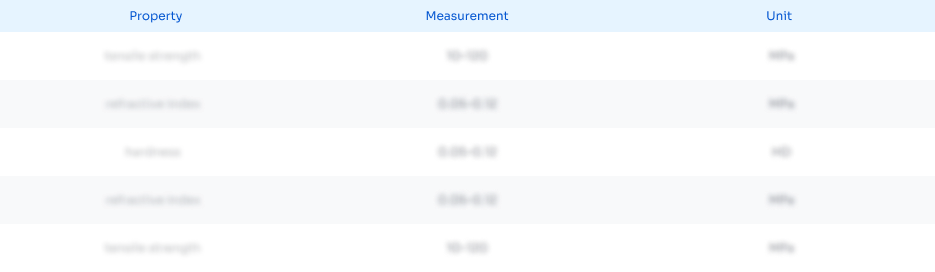
Abstract
Description
Claims
Application Information
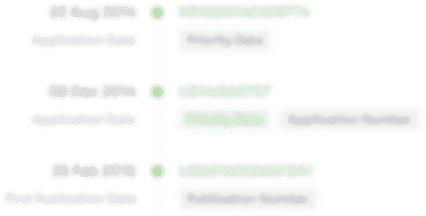
- R&D
- Intellectual Property
- Life Sciences
- Materials
- Tech Scout
- Unparalleled Data Quality
- Higher Quality Content
- 60% Fewer Hallucinations
Browse by: Latest US Patents, China's latest patents, Technical Efficacy Thesaurus, Application Domain, Technology Topic, Popular Technical Reports.
© 2025 PatSnap. All rights reserved.Legal|Privacy policy|Modern Slavery Act Transparency Statement|Sitemap|About US| Contact US: help@patsnap.com